Instrument pointer jittering recognition method based on support vector machine during instrument detection
A technology of support vector machine and instrument pointer, applied in character and pattern recognition, instruments, computer parts and other directions, can solve the problems of inaccurate recognition accuracy, pointer error, etc., to achieve short recognition time, improve accuracy, Improve real-time effect
- Summary
- Abstract
- Description
- Claims
- Application Information
AI Technical Summary
Problems solved by technology
Method used
Image
Examples
specific Embodiment approach 1
[0024] Specific Embodiment 1: A recognition method based on a support vector machine-based instrument pointer shake in instrument detection in this embodiment is specifically prepared according to the following steps:
[0025] Step 1. Divide the detected instrument types into various instrument subcategories;
[0026] Step 2. Obtain a clear picture of the instrument panel under normal conditions as a training sample before testing each instrument subcategory;
[0027] Step 3, preprocessing the training sample; wherein, the preprocessing refers to zooming the training sample image and using a noise suppression filter to perform denoising processing; if it is a grayscale image, then binarize the grayscale image ; Common noises include salt and pepper noise and Gaussian noise. The characteristic of salt and pepper noise is that the location of the noise is random, but the amplitude of the noise is basically the same; the characteristic of Gaussian noise is that the location is ce...
specific Embodiment approach 2
[0033] Specific embodiment 2: The difference between this embodiment and specific embodiment 1 is that in step 4, feature extraction is performed on the preprocessed image to obtain the feature space formed by each feature value of the image as follows:
[0034] (1) The extracted color, grayscale, contour and position feature values, extracting contour features to determine the position of the pointer vertex and the width of the pointer;
[0035] (2) Normalize the feature space formed by the extracted eigenvalues in Matlab; normalization can facilitate subsequent data processing, and speed up the convergence of the program when running, preventing network training caused by the existence of singular sample data Time increases and may cause the network to fail to converge; the normalized feature space is the training model. Other steps and parameters are the same as those in Embodiment 1.
specific Embodiment approach 3
[0036] Specific embodiment three: the difference between this embodiment and specific embodiment one or two is: in step five, according to the image feature space obtained in step four, the SVM classifier is used for training, and the specific process of generating the SVM training model of the training set is:
[0037] (1) The SVM model improves the generalization ability of the learning machine by seeking the minimum structural risk, and realizes the minimization of the empirical risk and the confidence range, so as to achieve the purpose of obtaining good statistical laws even when the statistical sample size is small; The goal of using the training data set to train the SVM model is to determine a dividing line to completely separate the two types of training samples with the label -1 and the label 1 in the training data set, so as to distinguish whether the pixel in the image is located between the instrument pointer Middle; the boundary line has different forms for differ...
PUM
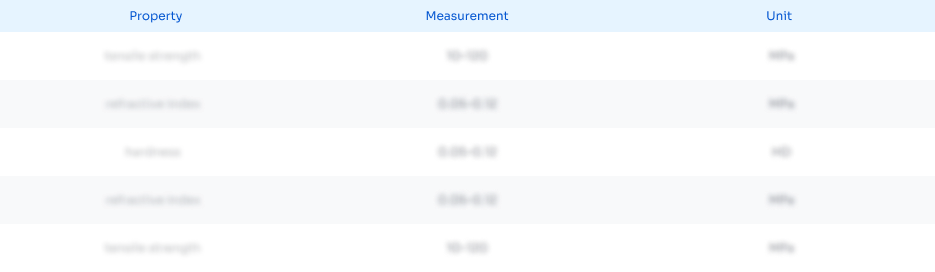
Abstract
Description
Claims
Application Information
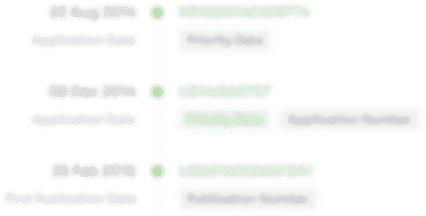
- R&D Engineer
- R&D Manager
- IP Professional
- Industry Leading Data Capabilities
- Powerful AI technology
- Patent DNA Extraction
Browse by: Latest US Patents, China's latest patents, Technical Efficacy Thesaurus, Application Domain, Technology Topic.
© 2024 PatSnap. All rights reserved.Legal|Privacy policy|Modern Slavery Act Transparency Statement|Sitemap