Multi-level-point-set characteristic extraction method applicable to ground laser radar point cloud classification
A terrestrial lidar, multi-level technology, applied in the field of spatial information, can solve problems such as noise data, missing, uneven point cloud density, etc.
- Summary
- Abstract
- Description
- Claims
- Application Information
AI Technical Summary
Problems solved by technology
Method used
Image
Examples
Embodiment 1
[0087] The performance of the present invention is verified qualitatively and quantitatively by using point cloud data of three urban scenes. The point clouds of these three scenes are obtained by single-station scanning of ground lidar, and the main objects in the scenes include buildings, trees, people and cars. The scene range is large, and the corresponding point density changes greatly. A small tree nearby often contains hundreds of thousands of points, while a tall building in the distance contains only a few thousand points. Single-station scanning can only obtain the surface data of the object facing the scanner, and the rear object is often blocked by the front object, resulting in data loss. To train these classifiers and evaluate the present invention, the three scenarios were identified manually, and the identified results were taken as ground truth.
[0088] From aspects such as the accuracy of the learning process and classification process, the present inventio...
PUM
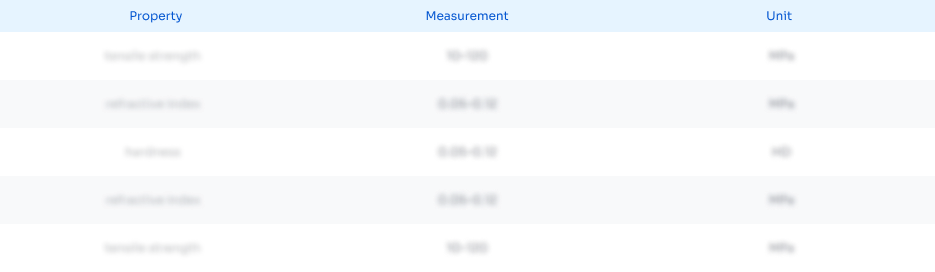
Abstract
Description
Claims
Application Information
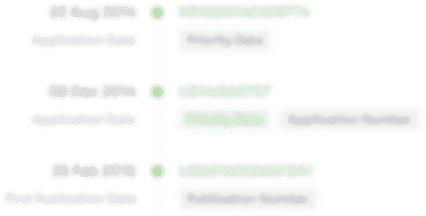
- R&D Engineer
- R&D Manager
- IP Professional
- Industry Leading Data Capabilities
- Powerful AI technology
- Patent DNA Extraction
Browse by: Latest US Patents, China's latest patents, Technical Efficacy Thesaurus, Application Domain, Technology Topic, Popular Technical Reports.
© 2024 PatSnap. All rights reserved.Legal|Privacy policy|Modern Slavery Act Transparency Statement|Sitemap|About US| Contact US: help@patsnap.com