Cluster-feature-weighted fuzzy compact scattering and clustering method
A technology of feature weighting and clustering method, applied in the field of data processing, can solve the problems of unbalanced data division of sample distribution, failure to consider the actual situation of hard division of samples, and no consideration of boundary points of hard division, and achieve high clustering accuracy, The effect of reducing time-consuming and good clustering performance
- Summary
- Abstract
- Description
- Claims
- Application Information
AI Technical Summary
Problems solved by technology
Method used
Image
Examples
Embodiment 1
[0098] In order to better illustrate the performance of the present invention, we adopt the method of the present invention to carry out classification experiments for one of the real data sets of UCI repository of machine learning databases: the Iris data set, and the fuzzy index m is respectively set to (1.5,2,2.5,3 ,3.5), the iteration error precision is 10 -6 , the parameters β in the cluster feature addition algorithm CWFCS algorithm of the present invention are respectively set to (0.005, 0.05, 0.5, 1), in order to represent the unbalanced distribution of samples, the Iris data set retains all the data of the first and second classes and uses them from the third Randomly select 10 samples from the class, and a total of 110 samples are divided into 3 categories, wherein the 2nd category and the 3rd category have intersections, and the clustering results using the algorithm of the present invention (abbreviated as CWFCS algorithm) are as follows Figure 2 ~ Figure 6 shown....
Embodiment 2
[0101] In order to verify the superiority of the present invention, we use FCS, WFCM and the CWFCS provided by the present invention to test the Iris data set respectively.
[0102] In the experiment, the fuzzy index m in the experiment is set to (1.5, 2, 2.5, 3, 3.5) error! Reference source not found. , the iteration error precision is 10 -6 , the parameters β in the CWFCS algorithm are respectively set to (0.005, 0.05, 0.5, 1); the experiment is repeated 100 times, and the optimal result and the average result are taken. Use the accuracy rate (Accuracy), the number of iterations (Iter), and the execution time (Time) to measure the optimal performance of the algorithm, and use the average accuracy rate (avg_Accuracy, the number of samples correctly divided / total number of samples), the average number of iterations (avg_Iter) and the average execution time (avg_Time) to measure the overall performance of the algorithm. The best and average results of the clustering results o...
Embodiment 3
[0111] We then use FCS, WFCM and the three methods of CWFCS provided by the present invention to experiment on the Breast Cancer data set. The Breast Cancer data set has 30 attributes in total. In order to represent the unbalanced distribution of samples, the first type randomly selects 10 samples, and the first type randomly selects 10 samples. There are 367 samples in the second category, and the results are shown in Table 2. Table 3 shows that the performance of CWFCS algorithm is the most stable, the number of iterations is slightly higher than that of WFCM algorithm, the execution time is within 0.1 seconds, and the clustering accuracy is higher than the other two algorithms.
[0112] Algorithms
[0113] table 3
PUM
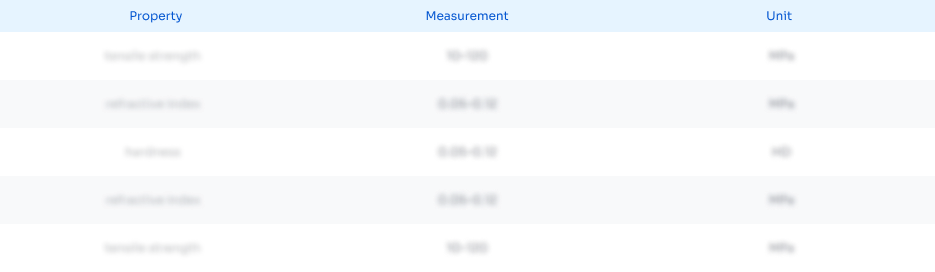
Abstract
Description
Claims
Application Information
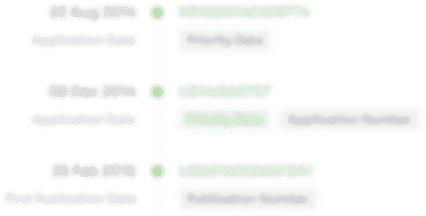
- R&D
- Intellectual Property
- Life Sciences
- Materials
- Tech Scout
- Unparalleled Data Quality
- Higher Quality Content
- 60% Fewer Hallucinations
Browse by: Latest US Patents, China's latest patents, Technical Efficacy Thesaurus, Application Domain, Technology Topic, Popular Technical Reports.
© 2025 PatSnap. All rights reserved.Legal|Privacy policy|Modern Slavery Act Transparency Statement|Sitemap|About US| Contact US: help@patsnap.com