Layering single-class ship target false alarm eliminating method based on intra-class difference
A technology of false alarm elimination and intra-class difference, which is applied in the field of target recognition, can solve the problems of difficult generalization and fitting, effective elimination of false alarms of ships, and rapid output of unfavorable judgment targets, so as to ensure detection performance, The effect of good promotion performance
- Summary
- Abstract
- Description
- Claims
- Application Information
AI Technical Summary
Problems solved by technology
Method used
Image
Examples
Embodiment
[0074] In this example, 1712 large ship slices, 1688 small ship slices, and 74406 false alarm slices were selected for experimentation. Take half of each as the training set, respectively: RDC1, RXC1, RXJ1, and the remaining half as the prediction set, respectively: RDC2, RXC2, RXJ2.
[0075] Step 1: Perform feature extraction on all large ships, small ships, and false alarm slices to obtain 17-dimensional feature vectors.
[0076] Step 2: Use half of the large ship data set RDC1 as the training set for parameter optimization, select the parameter group under the corresponding average detection probability according to actual needs, and use RDC1 to train the generated classifier 1.
[0077] The third step: Use the classifier 1 obtained in the second step to predict RDC1, RXC1, and RXJ1 to obtain a large ship error diversity set EDC1, a small ship error diversity set EXC1, and a false alarm rejection set EXJ1.
[0078] Step 4: Use the big boat error classification EDC1 to opti...
PUM
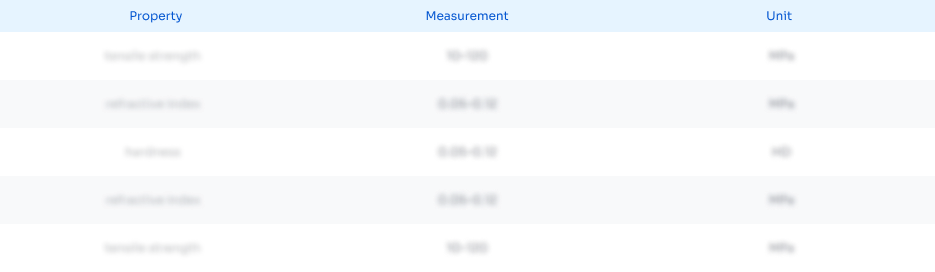
Abstract
Description
Claims
Application Information
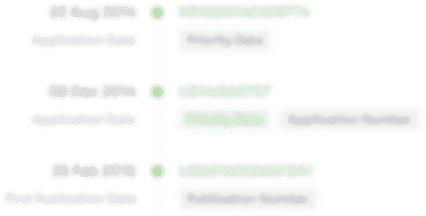
- R&D Engineer
- R&D Manager
- IP Professional
- Industry Leading Data Capabilities
- Powerful AI technology
- Patent DNA Extraction
Browse by: Latest US Patents, China's latest patents, Technical Efficacy Thesaurus, Application Domain, Technology Topic, Popular Technical Reports.
© 2024 PatSnap. All rights reserved.Legal|Privacy policy|Modern Slavery Act Transparency Statement|Sitemap|About US| Contact US: help@patsnap.com