Automatic market analysis method based on multi-mode learning
A market analysis and multi-modal technology, applied in marketing and other directions, can solve problems such as risk increase, market change grasp, market change error prediction, etc., to improve performance and accuracy, reduce risk, and have strong applicability Effect
- Summary
- Abstract
- Description
- Claims
- Application Information
AI Technical Summary
Problems solved by technology
Method used
Examples
Embodiment 1
[0022] Embodiment 1, a kind of automatic market analysis method based on multimodal learning, first trains the learning device, and then utilizes the trained learning device to predict the market in actual use; the method for the training of the learning device is: first Collect information of different modalities such as market data or market description text information and mark the information; then use the multi-instance generation method of market data features and text features to convert the underlying features into the form of multi-instance packages; finally adopt the Using a variety of multi-instance multi-label learning methods of different modalities to fuse data and perform multi-label learning.
Embodiment 2
[0023] Embodiment 2, in the automatic market analysis method based on multimodal learning described in Embodiment 1: the specific steps of the training of the learning device are as follows:
[0024] Step 100, collect various modal information of the market, and manually mark the collected objects;
[0025] Step 101, convert the collected underlying features of market information into a multi-instance package representation through the multi-instance generation method: {(x, t), y}, where the media object is marked as x, and the corresponding other modal information is recorded as t , manually marked as y;
[0026] Step 102, use the training model M to train the collected data to obtain relevant model parameters: marker generation sub-model parameters α, β y ;Market direct market eigenmode information generation sub-model parameter β c ;Other modal information generation sub-model parameters β t And the multimodal input latent variable controls the model parameter η.
[002...
Embodiment 3
[0032] Embodiment 3, in the automatic market analysis method based on multimodal learning described in embodiment 1 and embodiment 2: the specific steps of the training of the learning device are as follows: the steps of using the learning device are as follows:
[0033] Step 200, collect test market data characteristics (if there are other modal data, also collect);
[0034] Step 201, transform the underlying characteristics of the market conditions into the representation form {(x)} or {(x, t)} of the multi-instance package through the multi-instance generation method;
[0035] Step 202, use the training model M to process the new market feature I, and output the prediction mark y.
[0036] The generative probability model modeling method of the training model M is:
[0037] (1) (marker-theme submodel part) Let the market quotations be generated by the theme model, where the mark y is determined by the parameter α through the Latent Dirichlet Allocation (LDA) submodel and t...
PUM
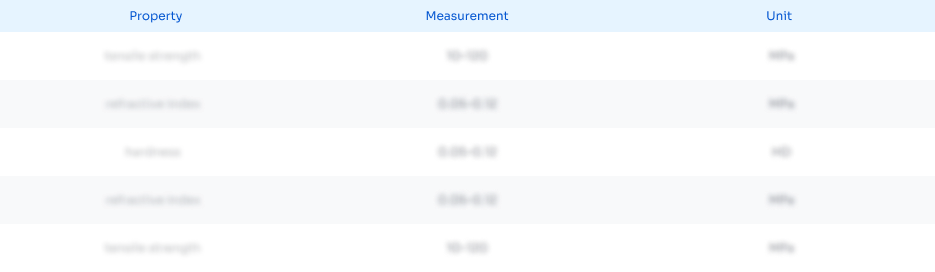
Abstract
Description
Claims
Application Information
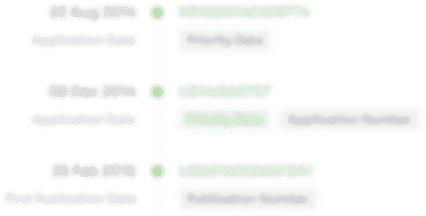
- R&D Engineer
- R&D Manager
- IP Professional
- Industry Leading Data Capabilities
- Powerful AI technology
- Patent DNA Extraction
Browse by: Latest US Patents, China's latest patents, Technical Efficacy Thesaurus, Application Domain, Technology Topic.
© 2024 PatSnap. All rights reserved.Legal|Privacy policy|Modern Slavery Act Transparency Statement|Sitemap