Semi-supervised hierarchical clustering method based on ultrametric distance matrix
A technology of distance matrix and hierarchical clustering, applied in the field of clustering, can solve the problems of high time complexity of HAC, imprecise optimal number of clusters, limited effectiveness, etc.
- Summary
- Abstract
- Description
- Claims
- Application Information
AI Technical Summary
Problems solved by technology
Method used
Image
Examples
Embodiment Construction
[0031] combine image 3 , a semi-supervised hierarchical clustering method based on hypermetric distance matrix, including the following steps:
[0032] Step 1, define inequality constraints A closed convex set of , and will be C, E projected to in is an m*1 vector used to represent the n*n symmetric dissimilarity matrix D; C is an m*r dissimilarity matrix x 1,1 x 1,2 . . . x 1 , r x 2,1 x 2,2 ...
PUM
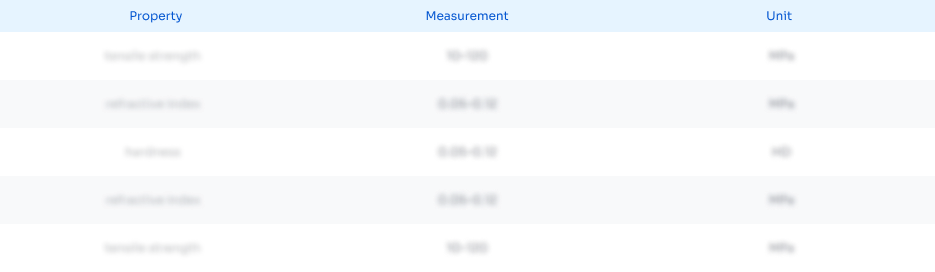
Abstract
Description
Claims
Application Information
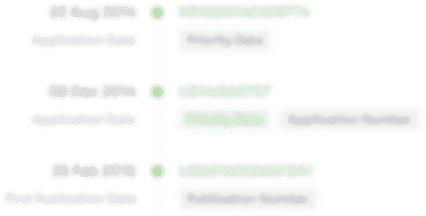
- R&D
- Intellectual Property
- Life Sciences
- Materials
- Tech Scout
- Unparalleled Data Quality
- Higher Quality Content
- 60% Fewer Hallucinations
Browse by: Latest US Patents, China's latest patents, Technical Efficacy Thesaurus, Application Domain, Technology Topic, Popular Technical Reports.
© 2025 PatSnap. All rights reserved.Legal|Privacy policy|Modern Slavery Act Transparency Statement|Sitemap|About US| Contact US: help@patsnap.com