Bearing fault classification diagnosis method based on sparse representation and LDM (large margin distribution machine)
A sparse representation and fault classification technology, applied in mechanical bearing testing, character and pattern recognition, instruments, etc., can solve the problems of incomplete signal decomposition, difficulty in maintaining observation signal characteristics, and low fault diagnosis accuracy.
- Summary
- Abstract
- Description
- Claims
- Application Information
AI Technical Summary
Problems solved by technology
Method used
Image
Examples
Embodiment Construction
[0105] The present invention proposes a bearing fault classification and diagnosis method based on sparse representation and large-interval distribution learning. The method realizes the transformation of signals from one-dimensional to high-dimensional through the Complete Ensemble Empirical Mode Decomposition (CEEMD) , which ensures the completeness of the decomposition, suppresses the modal aliasing phenomenon, and obtains a better decomposition effect. Aiming at the decomposed multi-dimensional sub-band information, a dimension reduction method based on sparse representation is introduced to complete the conversion from high dimension to target dimension, so that the reconstructed signal can better maintain the characteristics of the observed signal data. The source signal is separated by the eigenmatrix joint diagonalization blind source separation algorithm. Extract the time-frequency features of the separated source signal and historical fault signals, and use the Large...
PUM
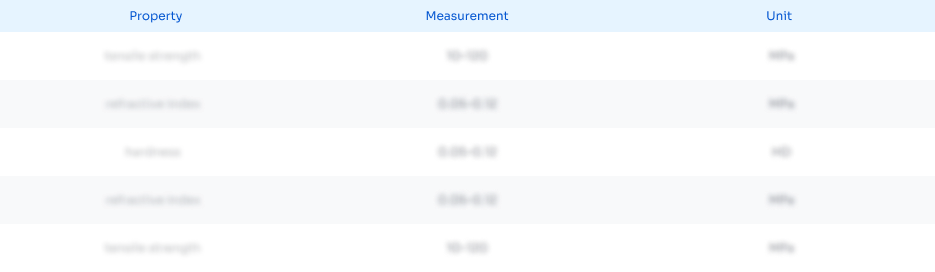
Abstract
Description
Claims
Application Information
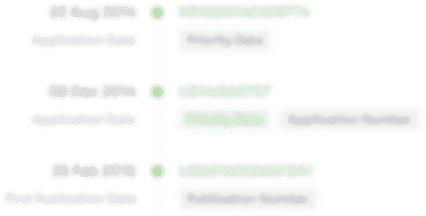
- R&D Engineer
- R&D Manager
- IP Professional
- Industry Leading Data Capabilities
- Powerful AI technology
- Patent DNA Extraction
Browse by: Latest US Patents, China's latest patents, Technical Efficacy Thesaurus, Application Domain, Technology Topic.
© 2024 PatSnap. All rights reserved.Legal|Privacy policy|Modern Slavery Act Transparency Statement|Sitemap