A Label-Free Time Series Anomaly Detection Method
A technology of category labeling and time series, applied in the field of time series anomaly detection, can solve the problems of manually setting the number of clusters in hierarchical clustering and unsatisfactory segmentation effect of fixed points of satellite telemetry data, so as to achieve compact and coupled segmentation results. high degree of effect
- Summary
- Abstract
- Description
- Claims
- Application Information
AI Technical Summary
Problems solved by technology
Method used
Image
Examples
specific Embodiment approach 1
[0036] Specific implementation mode one: combine figure 1To illustrate this embodiment, a time series anomaly detection method without category labels is specifically carried out according to the following steps:
[0037] Step 1. Segment the historical satellite telemetry data according to the periodic characteristics of the satellite telemetry data, and obtain a time series without class labels X={x 1 ,x 2 ,...,x n}, where n is a positive integer greater than 0, indicating the number of time series, x 1 is the first time series in the time series without category labels, x 2 is the second time series in the time series without class labels, x n is the nth time series in the time series without class labels;
[0038] Step 2. For the time series without class labels obtained in step 1, X={x 1 ,x 2 ,...,x n} Carry out adaptive hierarchical clustering, and determine and delete the abnormal sequence in the time series without category labels, and obtain the time series wi...
specific Embodiment approach 2
[0040] Specific embodiment 2: The difference between this embodiment and specific embodiment 1 is that in the step 1, the satellite telemetry historical data is segmented according to the periodic characteristics of the satellite telemetry data, and the time series X={x without category labels is obtained 1 ,x 2 ,...,x n}, where n is a positive integer greater than 0, indicating the number of time series, x 1 is the first time series in the time series without category labels, x 2 is the second time series in the time series without class labels, x n is the nth time series in the time series without class labels; the specific process is: segment the historical data of satellite telemetry with the point of change in argument as the mark, and obtain the time series without class labels X={x 1 ,x 2 ,...,x n}.
[0041] Argument is one of the test parameters in satellite telemetry data. Its value changes in order from 0 to 360. When it reaches 360, it becomes 0 and starts to ...
specific Embodiment approach 3
[0043] Specific embodiment three: the difference between this embodiment and specific embodiment one or two is: in the step two, the time series without class labels obtained in step one X={x 1 ,x 2 ,...,x n} Carry out adaptive hierarchical clustering, and determine and delete the abnormal sequence in the time series without category labels, and obtain the time series with category labels under the normal operation mode of the satellite and category labels where n z is a positive integer greater than 0, indicating the number of normal time series, x' 1 is the first normal time series in the time series with category labels, x' 2 is the second normal time series in the time series with class labels, is the nth time series with category labels z a normal time series, l' 1 is the first normal time series in the category label, l' 2 is the second normal time series in the category labels, is the nth in the category label z A normal time series; the specific process i...
PUM
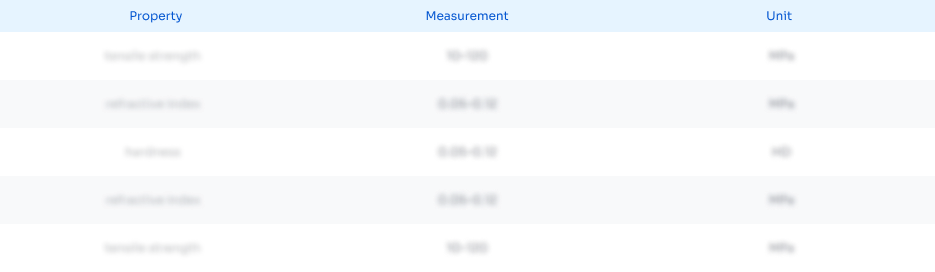
Abstract
Description
Claims
Application Information
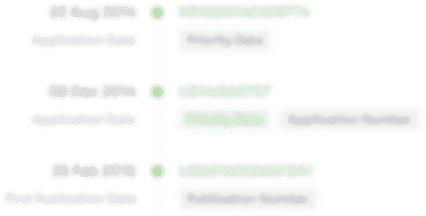
- R&D Engineer
- R&D Manager
- IP Professional
- Industry Leading Data Capabilities
- Powerful AI technology
- Patent DNA Extraction
Browse by: Latest US Patents, China's latest patents, Technical Efficacy Thesaurus, Application Domain, Technology Topic, Popular Technical Reports.
© 2024 PatSnap. All rights reserved.Legal|Privacy policy|Modern Slavery Act Transparency Statement|Sitemap|About US| Contact US: help@patsnap.com