Power grid optimal carbon energy composite flow obtaining method based on swarm intelligence reinforcement learning
A technology of reinforcement learning and acquisition method, which is applied in the field of acquisition of optimal carbon-energy composite flow of power grid based on swarm intelligence reinforcement learning, and can solve problems such as fusion of algorithm advantages
- Summary
- Abstract
- Description
- Claims
- Application Information
AI Technical Summary
Problems solved by technology
Method used
Image
Examples
Embodiment
[0062] This embodiment discloses a method for obtaining the optimal carbon energy compound flow of the power grid based on swarm intelligence reinforcement learning. This embodiment aims at the IEEE118 power grid load node system to obtain the optimal carbon energy compound flow of the power grid, wherein the IEEE118 power grid load node system includes 54 units and 186 branches, according to the "2006 IPCC Guidelines for National Greenhouse Gas Inventory", obtain the carbon emission intensity of each unit in the grid load node system. Such as figure 1 As shown, in this embodiment, the power grid load node system based on swarm intelligence reinforcement learning method for obtaining the optimal carbon-energy composite flow of the power grid is as follows:
[0063] S1. Construct a group intelligent reinforcement learning system according to the grid load node system, such as figure 2 Shown is a schematic diagram of the carbon-energy composite flow of the power grid load node...
PUM
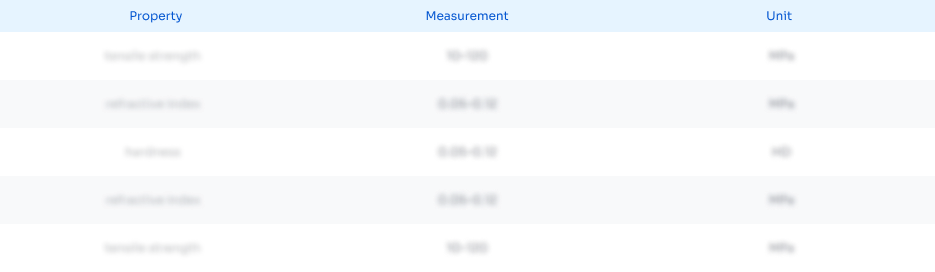
Abstract
Description
Claims
Application Information
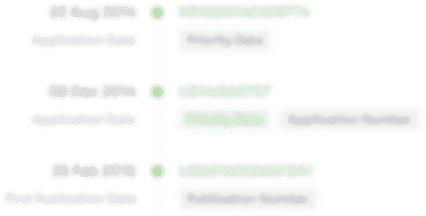
- R&D Engineer
- R&D Manager
- IP Professional
- Industry Leading Data Capabilities
- Powerful AI technology
- Patent DNA Extraction
Browse by: Latest US Patents, China's latest patents, Technical Efficacy Thesaurus, Application Domain, Technology Topic, Popular Technical Reports.
© 2024 PatSnap. All rights reserved.Legal|Privacy policy|Modern Slavery Act Transparency Statement|Sitemap|About US| Contact US: help@patsnap.com