Fluctuation wind speed prediction method based on extreme learning machine
A nuclear extreme learning machine, pulsating wind speed technology, applied in special data processing applications, instruments, electrical digital data processing and other directions, can solve the problems of model instability, difficult parameter setting of prediction models, etc. The effect of fixed height and strong generalization performance
- Summary
- Abstract
- Description
- Claims
- Application Information
AI Technical Summary
Problems solved by technology
Method used
Image
Examples
Embodiment Construction
[0030] The idea of the present invention is as follows: through ARMA numerical simulation to combine the pulsating wind speed samples with the new data-driven technology ELM, use numerical simulation to provide sample data for KELM simulation, and establish a learning mathematical model of extreme learning machine based on kernel function.
[0031] Below in conjunction with accompanying drawing, adopt the present invention to be described in further detail to single-point fluctuating wind speed prediction, the steps are as follows:
[0032] In the first step, the ARMA model is used to simulate and generate fluctuating wind speed samples at vertical spatial points, and the fluctuating wind speed samples at each spatial point are divided into two parts: training set and test set, and the training set and test set are normalized respectively, and the data After normalization processing, take the embedding dimension k=10 to perform phase space reconstruction on the sample data. ...
PUM
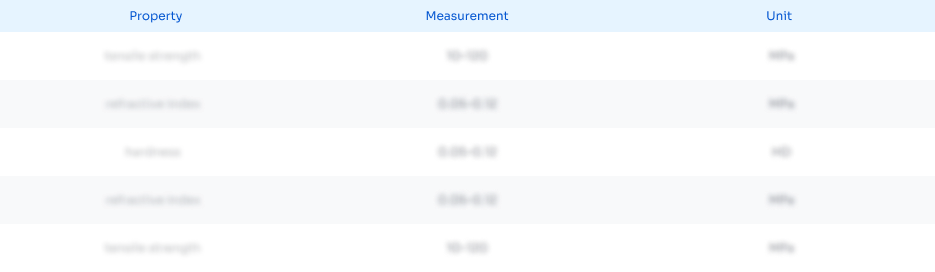
Abstract
Description
Claims
Application Information
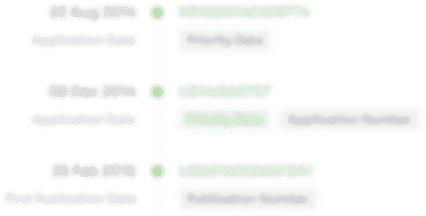
- R&D Engineer
- R&D Manager
- IP Professional
- Industry Leading Data Capabilities
- Powerful AI technology
- Patent DNA Extraction
Browse by: Latest US Patents, China's latest patents, Technical Efficacy Thesaurus, Application Domain, Technology Topic, Popular Technical Reports.
© 2024 PatSnap. All rights reserved.Legal|Privacy policy|Modern Slavery Act Transparency Statement|Sitemap|About US| Contact US: help@patsnap.com