Hybrid prediction method for network traffic
A network traffic and hybrid prediction technology, applied in the field of computer networks, can solve the problems that network traffic is difficult to ensure the accuracy of prediction, it is difficult to ensure the accuracy of prediction, and key parameters are difficult to determine.
- Summary
- Abstract
- Description
- Claims
- Application Information
AI Technical Summary
Problems solved by technology
Method used
Image
Examples
Embodiment Construction
[0052] The present invention will be described in further detail below in conjunction with the accompanying drawings.
[0053] Step 1: Collect network traffic data, divide the network traffic data into training set and test set, the training set is used for the establishment of ELM and ARIMA prediction models, and the test set is used to verify the prediction accuracy of the prediction model. Therefore, the network traffic sequence needs to be normalized, and the prediction results need to be denormalized to restore the real predicted value;
[0054] Step 2: Use the network traffic training sample sequence to train the ELM prediction model, use the experimental method to determine the embedding dimension m of the network traffic, that is, the number of ELM input layers, and determine the appropriate value by the root mean square of the error between the actual value and the ELM predicted value The embedding dimension m of . The ELM prediction process is as follows:
[0055] ...
PUM
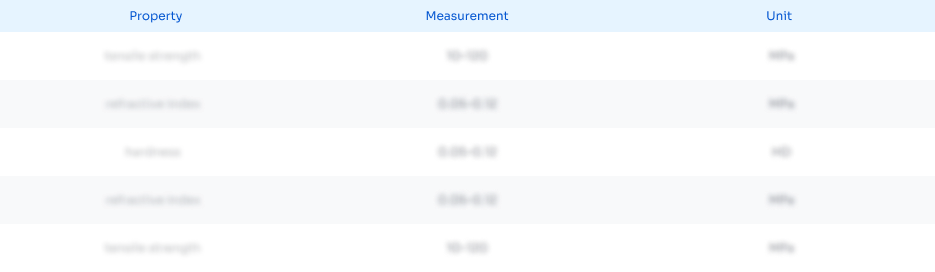
Abstract
Description
Claims
Application Information
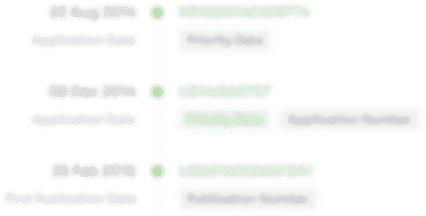
- R&D Engineer
- R&D Manager
- IP Professional
- Industry Leading Data Capabilities
- Powerful AI technology
- Patent DNA Extraction
Browse by: Latest US Patents, China's latest patents, Technical Efficacy Thesaurus, Application Domain, Technology Topic, Popular Technical Reports.
© 2024 PatSnap. All rights reserved.Legal|Privacy policy|Modern Slavery Act Transparency Statement|Sitemap|About US| Contact US: help@patsnap.com