Random forest parallelization machine studying method for big data in Spark cloud service environment
A random forest and machine learning technology, applied in the computer field, can solve the problems such as the performance degradation of the classification method and the long time, and achieve the effect of reducing the amount of calculation and complexity, reducing the impact, and improving the classification accuracy.
- Summary
- Abstract
- Description
- Claims
- Application Information
AI Technical Summary
Problems solved by technology
Method used
Image
Examples
Embodiment 1
[0038](1) Aiming at the problem that big data has high-dimensional features, the method of feature importance analysis is used in the training process and prediction process to reduce the dimensionality of high-dimensional data features, which effectively reduces the amount of calculation and complexity of the method. Aiming at the problem of a large amount of noisy data in big data, the weighted voting method is used for data set prediction and voting, which reduces the impact of noisy data on data classification voting results, and improves the classification accuracy of random forest machine learning methods for complex big data.
[0039] Step 1: The feature selection process of the training data during the random forest model training process, the process is as follows figure 1 shown. The specific implementation steps are as follows:
[0040] Step 1.1: Sampling the high-dimensional big data training set with replacement into n training data subsets;
[0041] Step 1.2: Ca...
PUM
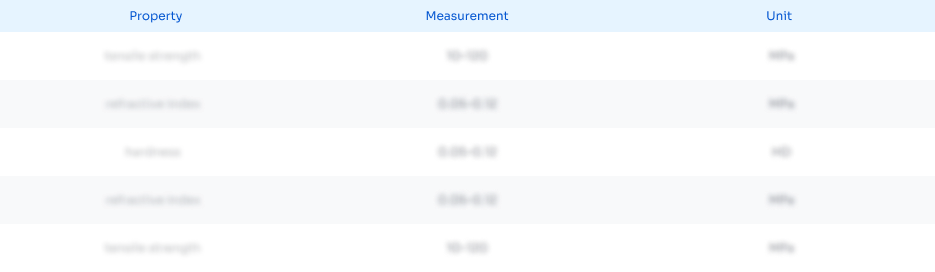
Abstract
Description
Claims
Application Information
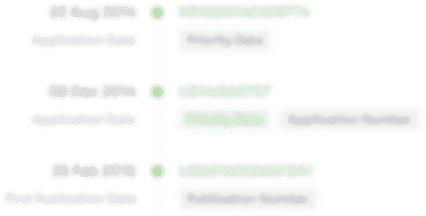
- R&D
- Intellectual Property
- Life Sciences
- Materials
- Tech Scout
- Unparalleled Data Quality
- Higher Quality Content
- 60% Fewer Hallucinations
Browse by: Latest US Patents, China's latest patents, Technical Efficacy Thesaurus, Application Domain, Technology Topic, Popular Technical Reports.
© 2025 PatSnap. All rights reserved.Legal|Privacy policy|Modern Slavery Act Transparency Statement|Sitemap|About US| Contact US: help@patsnap.com