Fault diagnosis method during industrial process
A fault diagnosis and industrial process technology, applied in the direction of instruments, test/monitoring control systems, control/regulation systems, etc., can solve problems such as inability to guarantee the accuracy of diagnostic results, difficulty in obtaining them, pollution, etc.
- Summary
- Abstract
- Description
- Claims
- Application Information
AI Technical Summary
Problems solved by technology
Method used
Image
Examples
Embodiment 1
[0092] In embodiment 1, the Monte Carlo (MonteCarlo) numerical simulation instance is specifically described as:
[0093] x * = x 1 x 2 x 3 x 4 x 5 x 6 = - 0.1681 0.2870 - 0.2...
Embodiment 2
[0108] Embodiment 2 adopts the same training set of 3000 normal samples as in Embodiment 1, and changes the fault direction vector and fault amplitude of the fault samples to be tested, namely ξ 4 =[000100] T , f 4 =10 -5 ×q 2 , (q=1,2,...1000), a total of 1000 fault samples to be tested are generated. The specific process of carrying out fault diagnosis to embodiment 2 is the same as embodiment 1, image 3 (a), image 3 (b), image 3 (c) respectively shows the diagnosis results of the contribution graph method, the method based on the maximum a posteriori probability and the fault diagnosis method provided by the present invention in Embodiment 2.
[0109] Figure 4 (a) and Figure 4 (b) shows the different fluctuation ranges of the feature value when the process variable is selected by the reconstruction contribution value and the relative reconstruction contribution value respectively; Figure 4 (a) is the nRBC i (x t ) as a feature quantity, the value of the fea...
PUM
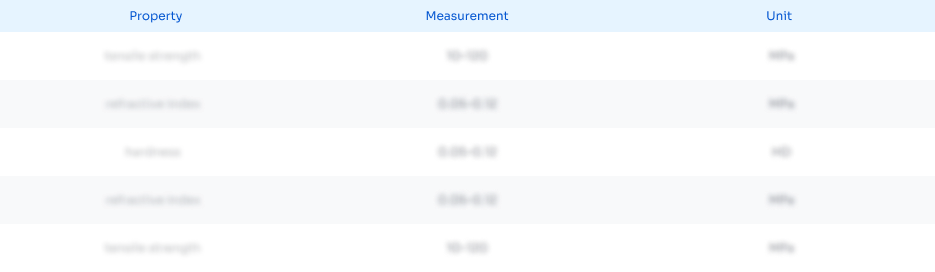
Abstract
Description
Claims
Application Information
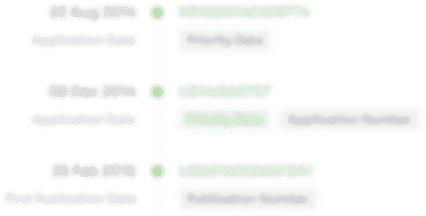
- R&D Engineer
- R&D Manager
- IP Professional
- Industry Leading Data Capabilities
- Powerful AI technology
- Patent DNA Extraction
Browse by: Latest US Patents, China's latest patents, Technical Efficacy Thesaurus, Application Domain, Technology Topic, Popular Technical Reports.
© 2024 PatSnap. All rights reserved.Legal|Privacy policy|Modern Slavery Act Transparency Statement|Sitemap|About US| Contact US: help@patsnap.com