Lung cancer image fine classification method based on fusion of LBP and wavelet moment features
A technology of fine classification and fusion of features, which is applied in character and pattern recognition, instruments, computing, etc., can solve the problems of lack of research on image fine classification in the medical field, and achieve the effects of improving classification accuracy, comprehensively representing images, and good recognition effects
- Summary
- Abstract
- Description
- Claims
- Application Information
AI Technical Summary
Problems solved by technology
Method used
Image
Examples
Embodiment Construction
[0031] Step 1: Use the lesion area detection method based on the gray level change to locate the lesion on the original input image.
[0032] In the template matching framework proposed before, the first step adopts the method of randomly generating a large number of templates. Before generating the templates, no preprocessing is performed, resulting in a large number of redundant template blocks containing useless information. In the subsequent scaling and matching process, the extracted features If the dimension is too large, the classification accuracy will decrease. Based on this, before generating the template block, we use the lesion area detection method based on the gray level change to locate the lesion first. figure 2 Because the image texture reflects a local structural feature of the image, which is specifically manifested as a certain change in the gray level or color of pixels in a certain neighborhood of the image, it can be used to quantitatively describe the ...
PUM
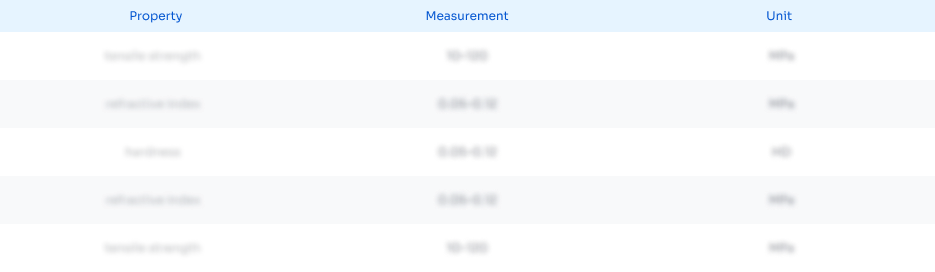
Abstract
Description
Claims
Application Information
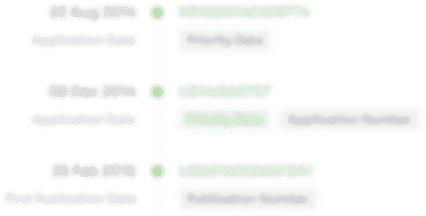
- R&D
- Intellectual Property
- Life Sciences
- Materials
- Tech Scout
- Unparalleled Data Quality
- Higher Quality Content
- 60% Fewer Hallucinations
Browse by: Latest US Patents, China's latest patents, Technical Efficacy Thesaurus, Application Domain, Technology Topic, Popular Technical Reports.
© 2025 PatSnap. All rights reserved.Legal|Privacy policy|Modern Slavery Act Transparency Statement|Sitemap|About US| Contact US: help@patsnap.com