Multi-time scale electric vehicle clustering schedulable capacity prediction method
A multi-time scale, electric vehicle technology, applied in electric vehicles, electric vehicle charging technology, forecasting, etc., can solve the problem of not considering multi-time scale power scheduling, low accuracy of probability models, and not considering dispatchable capacity calculation and prediction, etc. question
- Summary
- Abstract
- Description
- Claims
- Application Information
AI Technical Summary
Problems solved by technology
Method used
Image
Examples
Embodiment Construction
[0062] Multi-time scale electric vehicle cluster dispatchable capacity prediction methods include real-time electric vehicle cluster dispatchable capacity prediction and day-ahead electric vehicle cluster dispatchable capacity prediction, electric vehicle cluster dispatchable capacity includes electric vehicle cluster dispatchable charging capacity and electric vehicle cluster dispatchable capacity discharge capacity.
[0063] In this embodiment, the prediction method is carried out according to the following steps:
[0064] Step 1. Set the forecast time scale t for real-time electric vehicle cluster dispatchable capacity forecast d ; The time scale based on power system real-time scheduling is usually 1 second to 10 minutes, so t d The value ranges from 1 second to 10 minutes; to predict the time scale t d is the time interval to collect the real-time status data of electric vehicles connected to the grid area; t d The smaller the value, the more accurate the real-time ele...
PUM
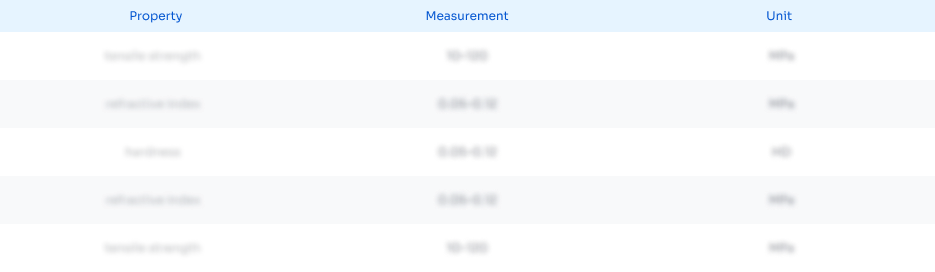
Abstract
Description
Claims
Application Information
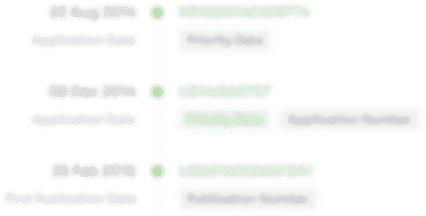
- R&D Engineer
- R&D Manager
- IP Professional
- Industry Leading Data Capabilities
- Powerful AI technology
- Patent DNA Extraction
Browse by: Latest US Patents, China's latest patents, Technical Efficacy Thesaurus, Application Domain, Technology Topic, Popular Technical Reports.
© 2024 PatSnap. All rights reserved.Legal|Privacy policy|Modern Slavery Act Transparency Statement|Sitemap|About US| Contact US: help@patsnap.com