Vehicle type recognition method based on rapid R-CNN deep neural network
A technology of deep neural network and vehicle identification, applied in the field of vehicle identification based on fast R-CNN deep neural network, can solve the problems of reducing training and learning time
- Summary
- Abstract
- Description
- Claims
- Application Information
AI Technical Summary
Problems solved by technology
Method used
Image
Examples
Embodiment 1
[0120] refer to Figure 1-14 , the technical solution adopted by the present invention to solve its technical problems is:
[0121] (1) About designing a fast visual segmentation algorithm for vehicle objects;
[0122] First, a fast visual segmentation algorithm for vehicle objects is designed, that is, region selection and localization of vehicle objects are performed;
[0123] In order to locate the position of the vehicle target; since the vehicle target may appear in any position of the image, and the size and aspect ratio of the target are also uncertain, the original technology initially uses a sliding window strategy to traverse the entire image, And it is necessary to set different scales and different aspect ratios; although this exhaustive strategy includes all possible positions of the target, the disadvantages are also obvious: the time complexity is too high, and too many redundant windows are generated, which is also Seriously affect the speed and performance o...
Embodiment 2
[0246] The visual recognition technology of the present invention has universality and is suitable for subclass recognition of other objects. As long as the data participating in the training is run in the system developed by the present invention to learn, and the characteristics of this type of object can be obtained, the recognition of this type of object can be realized. Subclass recognition task.
Embodiment 3
[0248] The visual recognition technology of the present invention has expansibility. After a new subclass appears, it is not necessary to relearn and train the original trained network features, as long as the new subclass is trained and learned, and the softmax classifier in the system is extended categorized data.
PUM
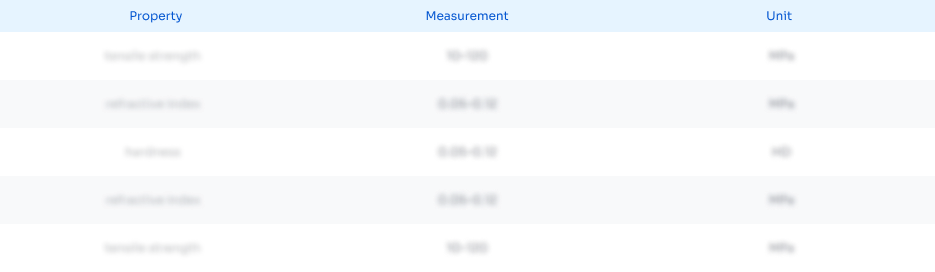
Abstract
Description
Claims
Application Information
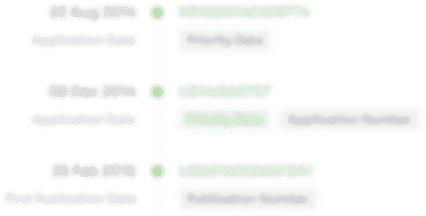
- Generate Ideas
- Intellectual Property
- Life Sciences
- Materials
- Tech Scout
- Unparalleled Data Quality
- Higher Quality Content
- 60% Fewer Hallucinations
Browse by: Latest US Patents, China's latest patents, Technical Efficacy Thesaurus, Application Domain, Technology Topic, Popular Technical Reports.
© 2025 PatSnap. All rights reserved.Legal|Privacy policy|Modern Slavery Act Transparency Statement|Sitemap|About US| Contact US: help@patsnap.com