Hybrid Gauss model matching method based on improved soil moving distance
A technology of mixed Gaussian model and matching method, which is applied in the field of efficient and robust measurement algorithm, can solve the problems of calculation efficiency and noise sensitivity, and achieve the effect of wide application prospect and accurate and efficient calculation
- Summary
- Abstract
- Description
- Claims
- Application Information
AI Technical Summary
Problems solved by technology
Method used
Image
Examples
Embodiment Construction
[0019] The invention proposes an improved bulldozing distance algorithm system based on a deep convolutional neural network. The core of the technology is to use a deep convolutional neural network to extract image features and model the image through GMM, and to achieve the matching between GMMs through the I-EMD algorithm, in which the Riemannian geometric structure design of Gaussian distribution is used to measure the distance between Gaussian distributions . The specific implementation of the present invention comprises the following steps (the system flowchart and the schematic diagram on image classification are respectively as attached figure 1 , with figure 2 shown):
[0020] Step 1: Extract the deep convolutional neural network features of the image. Scale-transform the image to obtain images of 3 different scales, perform convolution operation on the 3-scale images with the 19-layer VGGNet trained in advance, and take the last layer of convolutional layer (16th ...
PUM
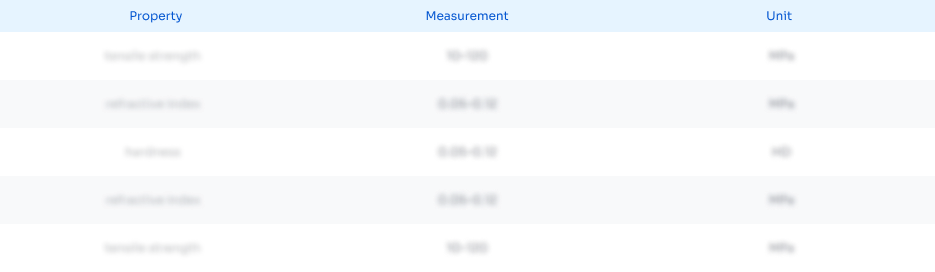
Abstract
Description
Claims
Application Information
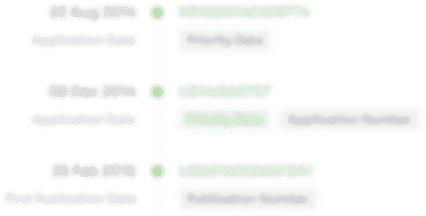
- R&D Engineer
- R&D Manager
- IP Professional
- Industry Leading Data Capabilities
- Powerful AI technology
- Patent DNA Extraction
Browse by: Latest US Patents, China's latest patents, Technical Efficacy Thesaurus, Application Domain, Technology Topic.
© 2024 PatSnap. All rights reserved.Legal|Privacy policy|Modern Slavery Act Transparency Statement|Sitemap