Improved LR-Bagging algorithm based on characteristic selection
A feature selection and algorithm technology, applied in computing, instrumentation, data processing applications, etc., can solve problems such as the small number of variables, the model is not direct enough, and the model prediction results are not satisfactory, so as to reduce the possibility and improve the diversity effect.
- Summary
- Abstract
- Description
- Claims
- Application Information
AI Technical Summary
Problems solved by technology
Method used
Image
Examples
Embodiment 1
[0034] This embodiment is based on the improved algorithm of this description to make predictions for Guiyang electric power arrears high-risk residential customers, combined with figure 2 The model establishment and solution process, the specific steps are as follows:
[0035] Step 1: Determine the initial data set from the original data, and the degree of correlation between the independent variable and the dependent variable cannot be too low;
[0036] Step 2: Perform WEO coding on discrete independent variables;
[0037] Step 3: Training and testing of the base LR model, integrating the combined model;
[0038] Step 4: Carry out the loop iteration of step 3 until the combination model is better;
[0039] Step 5: Prediction and evaluation using the optimal combination model.
[0040] Among them, step 1 is specifically described as follows:
[0041] The application data involved in the present invention comes from the arrears data of the grid residents in Guiyang City, ...
PUM
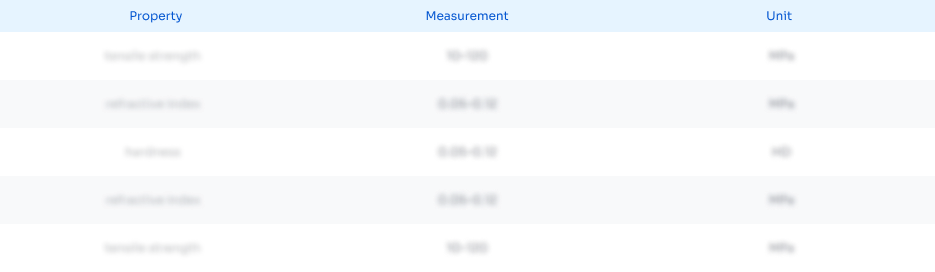
Abstract
Description
Claims
Application Information
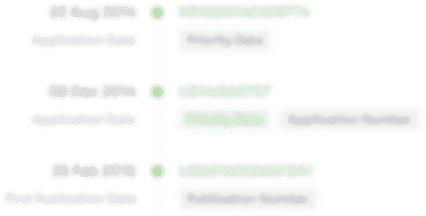
- R&D Engineer
- R&D Manager
- IP Professional
- Industry Leading Data Capabilities
- Powerful AI technology
- Patent DNA Extraction
Browse by: Latest US Patents, China's latest patents, Technical Efficacy Thesaurus, Application Domain, Technology Topic, Popular Technical Reports.
© 2024 PatSnap. All rights reserved.Legal|Privacy policy|Modern Slavery Act Transparency Statement|Sitemap|About US| Contact US: help@patsnap.com