Intra-abdominal adipose tissue segmentation method based on deep learning
A deep learning and abdominal fat technology, applied in the medical field, can solve the problem of time-consuming, reduce the incidence of errors, improve efficiency, and achieve good learning effects
- Summary
- Abstract
- Description
- Claims
- Application Information
AI Technical Summary
Problems solved by technology
Method used
Image
Examples
Embodiment Construction
[0037] The present invention will be described in detail below in conjunction with the accompanying drawings and specific embodiments. This embodiment is carried out on the premise of the technical solution of the present invention, and detailed implementation and specific operation process are given, but the protection scope of the present invention is not limited to the following embodiments.
[0038] Such as figure 1 As shown, the present invention provides a method for intra-abdominal fat segmentation based on deep learning, which specifically includes the following steps:
[0039] 1) Identification of abdominal fat pixels. Due to the special shape characteristics of the abdomen, the active contour model (Activecontour model) has developed many algorithms on the basis of it. The basic idea is to use continuous curves to express the target edge, and define an energy functional so that its independent variables include edge curves, segmentation The process is transformed i...
PUM
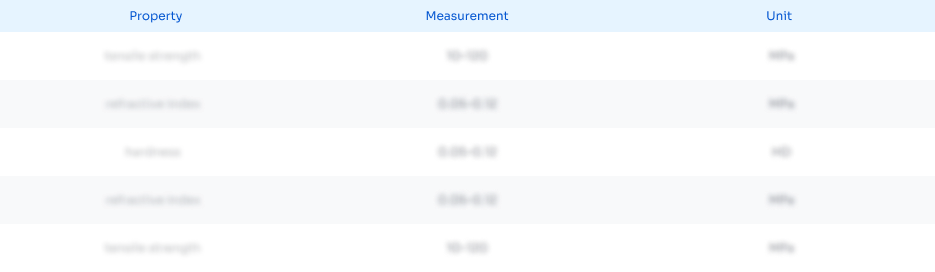
Abstract
Description
Claims
Application Information
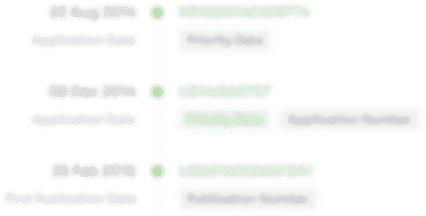
- Generate Ideas
- Intellectual Property
- Life Sciences
- Materials
- Tech Scout
- Unparalleled Data Quality
- Higher Quality Content
- 60% Fewer Hallucinations
Browse by: Latest US Patents, China's latest patents, Technical Efficacy Thesaurus, Application Domain, Technology Topic, Popular Technical Reports.
© 2025 PatSnap. All rights reserved.Legal|Privacy policy|Modern Slavery Act Transparency Statement|Sitemap|About US| Contact US: help@patsnap.com