Rapid area-of-interest detection method based on depth kernelized hashing
A region of interest and detection method technology, which is applied in the field of rapid detection and identification of medical breast image masses, can solve the problems of poor representation ability, overfitting, false detection rate or high false positive rate of medical images, and overcome the representation ability Insufficient, accurate output results, and the effect of reducing the amount of calculation
- Summary
- Abstract
- Description
- Claims
- Application Information
AI Technical Summary
Problems solved by technology
Method used
Image
Examples
Embodiment Construction
[0038] refer to figure 1 , the implementation of the present invention includes three parts: training, preprocessing and testing.
[0039] 1. Training part
[0040] Step 1, input image and label information.
[0041] In the image training set, randomly select two images as a set of image pairs, and combine all the images in the training set in pairs to form an image pair training set;
[0042] The image pair training set is divided into four categories, that is, the first category is the same label results and similar encoding results, the second category is the same label results but not similar encoding results, the third category is different label results but similar encoding results, and the fourth category is Classes are label results are different and encoding results are dissimilar.
[0043] Step 2, build a deep hash supervised learning network framework.
[0044] The deep hash supervised learning network framework is divided into ten layers, among which:
[0045] T...
PUM
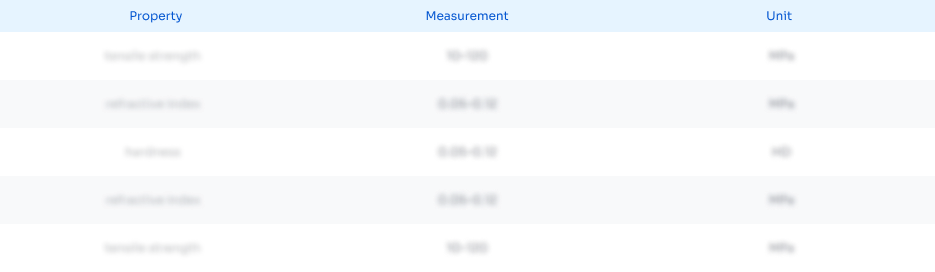
Abstract
Description
Claims
Application Information
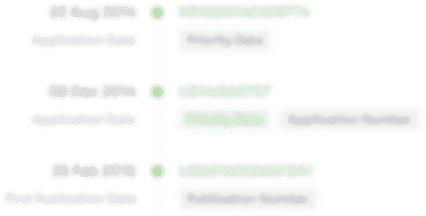
- R&D
- Intellectual Property
- Life Sciences
- Materials
- Tech Scout
- Unparalleled Data Quality
- Higher Quality Content
- 60% Fewer Hallucinations
Browse by: Latest US Patents, China's latest patents, Technical Efficacy Thesaurus, Application Domain, Technology Topic, Popular Technical Reports.
© 2025 PatSnap. All rights reserved.Legal|Privacy policy|Modern Slavery Act Transparency Statement|Sitemap|About US| Contact US: help@patsnap.com