Improved wavelet-analysis-based short-term power load prediction method
A technology of short-term power load and forecasting method, applied in forecasting, instrumentation, data processing applications, etc., can solve the problem of inability to accurately predict short-term power load, etc., to improve the level of load forecasting technology, save fuel and power generation costs, and improve economic benefits. and social benefits
- Summary
- Abstract
- Description
- Claims
- Application Information
AI Technical Summary
Problems solved by technology
Method used
Image
Examples
specific Embodiment approach 1
[0020] Specific implementation mode one: as figure 1 As shown, a short-term power load forecasting method based on wavelet analysis includes the following steps:
[0021] Step 1: Preprocessing the sample data;
[0022] Step 2: Select sample data;
[0023] Step 3: Normalize the sample data selected in Step 2;
[0024] Step 4: Establishing a wavelet regression analysis prediction model;
[0025] Step 5: Analysis and correction of forecast results.
specific Embodiment approach 2
[0026] Embodiment 2: The difference between this embodiment and Embodiment 1 is that the specific process of preprocessing the sample data in Step 1 is as follows:
[0027] Before using these sample data, process it, remove irregular data and fill in missing data, eliminate the influence of bad data or bad data, further data preprocessing can also reduce short-term power load intelligent forecasting method research and reduce input vector capacity , to avoid problems due to increased sample size and irregularities in the data;
[0028] Process the sample data, remove irregular data and fill in missing data. The change law of power system load data is expressed as:
[0029]
[0030] where Y is the true value of the load, is the observed value, V is the comprehensive action value of noise and random interference; let the mean value of V be Variance is σ 2 ; Select the threshold θ, when the observed value When the error value with the load true value Y is greater than θ...
specific Embodiment approach 3
[0032] Specific implementation mode three: the difference between this implementation mode and specific implementation mode one or two is: the specific process of selecting sample data in said step two is:
[0033] Use historical load data of similar days as historical load samples for forecasting. Therefore, the key to selecting historical load samples is the selection of similar days. The selection of similar days has a direct impact on the prediction accuracy. A similar day selection algorithm based on correlation factor mapping is adopted.
[0034] A similar day selection algorithm based on correlation factor mapping is adopted, which is divided into the following two parts:
[0035] (1) Calculation of the characteristic quantities of related factors between different days:
[0036] Introduce the quantitative index vector of each day, X i =[x i1 ,x i2 ,...,x im ], is the value of all feature quantities on the i-th day, assuming that there are m feature quantities in...
PUM
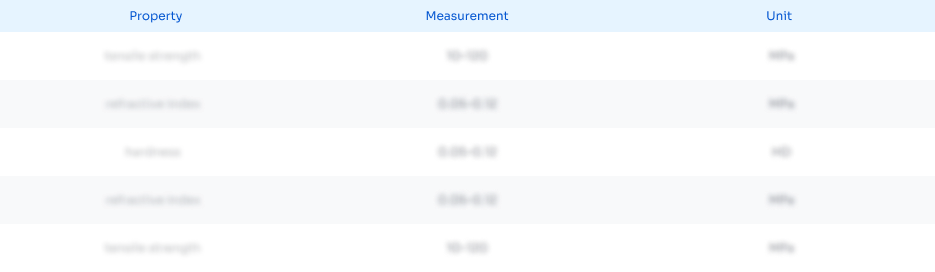
Abstract
Description
Claims
Application Information
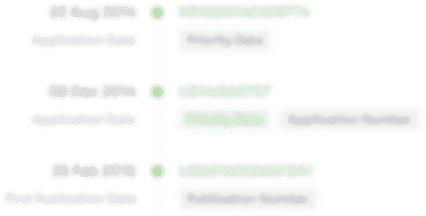
- R&D Engineer
- R&D Manager
- IP Professional
- Industry Leading Data Capabilities
- Powerful AI technology
- Patent DNA Extraction
Browse by: Latest US Patents, China's latest patents, Technical Efficacy Thesaurus, Application Domain, Technology Topic.
© 2024 PatSnap. All rights reserved.Legal|Privacy policy|Modern Slavery Act Transparency Statement|Sitemap