Domain Transfer Extreme Learning Machine Method Based on Manifold Regularization and Norm Regularization
A technology of extreme learning machine and domain, applied in the field of transfer extreme learning machine algorithm, which can solve the problems of data offset and lack of domain transfer ability.
- Summary
- Abstract
- Description
- Claims
- Application Information
AI Technical Summary
Problems solved by technology
Method used
Image
Examples
Embodiment Construction
[0046] In order to better understand the present invention, the present invention will be further described in detail below in conjunction with specific examples, but the following description is only for demonstration and explanation, and does not limit the present invention in any form.
[0047] The data used in this embodiment comes from the UCI machine learning database. The database contains data of 13,910 gas samples of 6 gases collected by an electronic nose system on a gas transmission platform for 36 consecutive months. In this embodiment, each sample is characterized by extracting a 128-dimensional feature. Since the gas detection sensor of the electronic nose system will have sensor drift with time, the collected gas data will also have data drift in different time periods. The flow process of the inventive method is as figure 1 shown.
[0048] Step 1: Take the data of 445 gas samples in the first and second months as the source field data of the sensorless drift...
PUM
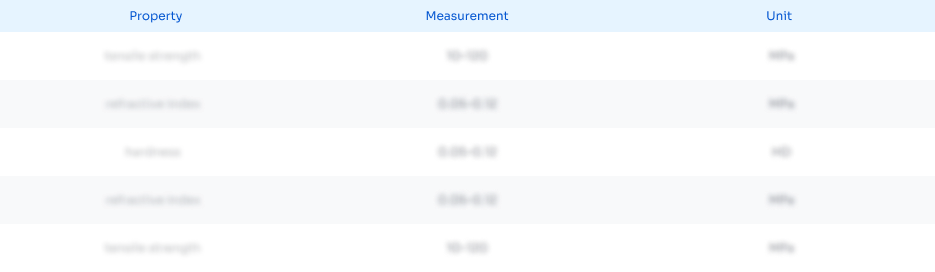
Abstract
Description
Claims
Application Information
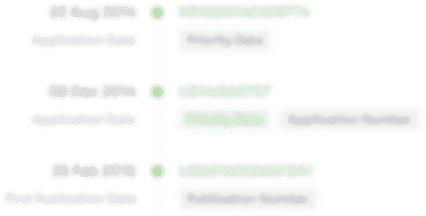
- R&D
- Intellectual Property
- Life Sciences
- Materials
- Tech Scout
- Unparalleled Data Quality
- Higher Quality Content
- 60% Fewer Hallucinations
Browse by: Latest US Patents, China's latest patents, Technical Efficacy Thesaurus, Application Domain, Technology Topic, Popular Technical Reports.
© 2025 PatSnap. All rights reserved.Legal|Privacy policy|Modern Slavery Act Transparency Statement|Sitemap|About US| Contact US: help@patsnap.com