Rolling bearing variable-work-condition fault diagnosis method based on visual cognition
A technology for rolling bearings and changing working conditions, which is applied in image data processing, instrumentation, electrical digital data processing, etc. It can solve the problems that STFT cannot meet the resolution and time, frequency confusion, and endpoint effects at the same time, and achieve the elimination of redundant fault characteristics. , high fault diagnosis accuracy, and the effect of improving calculation speed
- Summary
- Abstract
- Description
- Claims
- Application Information
AI Technical Summary
Problems solved by technology
Method used
Image
Examples
Embodiment Construction
[0053] The preferred embodiments of the present invention will be described in detail below in conjunction with the accompanying drawings. It should be understood that the preferred embodiments described below are only used to illustrate and explain the present invention, and are not intended to limit the present invention.
[0054] figure 1 It is a block diagram of a visual cognition-based fault diagnosis method for rolling bearings under variable working conditions provided by an embodiment of the present invention, as shown in figure 1 shown, including the following steps:
[0055] Using recursive graph technology to convert the vibration signal of rolling bearing under variable working conditions into a two-dimensional image;
[0056] Using the accelerated robust feature SURF algorithm to perform feature extraction on the two-dimensional image to obtain a high-dimensional fault feature vector with visual invariance;
[0057] Using an isometric mapping Isomap algorithm to...
PUM
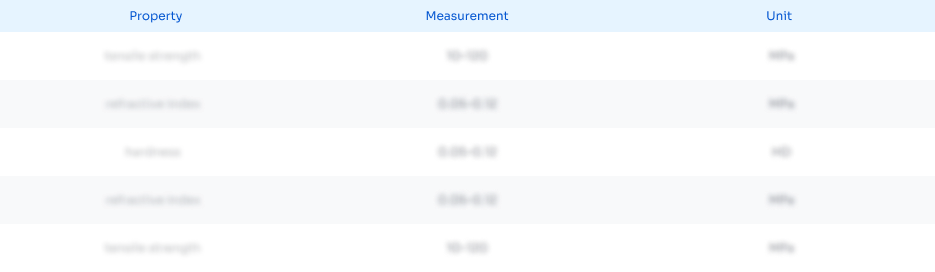
Abstract
Description
Claims
Application Information
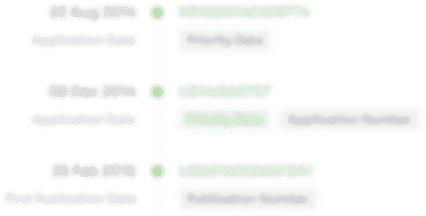
- R&D Engineer
- R&D Manager
- IP Professional
- Industry Leading Data Capabilities
- Powerful AI technology
- Patent DNA Extraction
Browse by: Latest US Patents, China's latest patents, Technical Efficacy Thesaurus, Application Domain, Technology Topic.
© 2024 PatSnap. All rights reserved.Legal|Privacy policy|Modern Slavery Act Transparency Statement|Sitemap