Sparse subspace clustering algorithm based on semi-supervision
A clustering algorithm and subspace technology, applied in computing, computer components, instruments, etc., can solve problems such as the inability to introduce semi-supervised frameworks, and achieve the effect of increasing applicability and improving clustering performance
- Summary
- Abstract
- Description
- Claims
- Application Information
AI Technical Summary
Problems solved by technology
Method used
Image
Examples
Embodiment Construction
[0029] specific implementation plan
[0030] The invention introduces the data prior information into the sparse subspace model, and establishes a semi-supervised framework on the sparse subspace clustering algorithm. Its specific process will be combined with figure 1 The overall flow chart of the algorithm shown is as follows:
[0031] Step 1: Establish a must-connected constraint matrix and a non-connected constraint matrix through the prior information of the data, the forms of which are shown in formula (1) and formula (2) respectively, and the establishment method has been explained in the summary of the invention.
[0032] Step 2: Introduce the data matrix Y and the constraint matrix MC, NC into the semi-supervised sparse subspace model, introduce the auxiliary matrix A and the Lagrange multiplier matrix Δ, and establish the Lagrang corresponding to the hard threshold or soft threshold form The daily augmentation function is shown in formula (5) and formula (6):
[0...
PUM
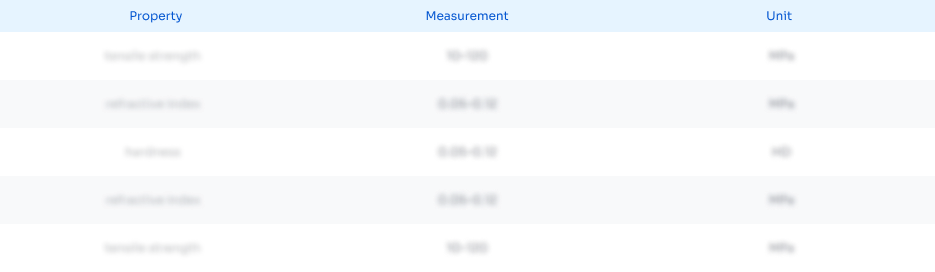
Abstract
Description
Claims
Application Information
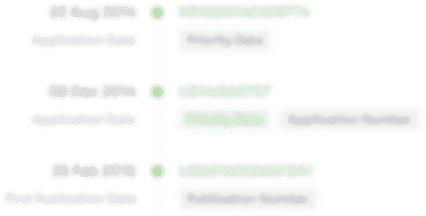
- R&D Engineer
- R&D Manager
- IP Professional
- Industry Leading Data Capabilities
- Powerful AI technology
- Patent DNA Extraction
Browse by: Latest US Patents, China's latest patents, Technical Efficacy Thesaurus, Application Domain, Technology Topic.
© 2024 PatSnap. All rights reserved.Legal|Privacy policy|Modern Slavery Act Transparency Statement|Sitemap