Convolution Neural Network (CNN) structure improving method
A network structure and convolutional neural technology, applied in the field of deep learning, can solve the problem of low training efficiency, achieve the effect of cumbersome operation process and improve network performance
- Summary
- Abstract
- Description
- Claims
- Application Information
AI Technical Summary
Problems solved by technology
Method used
Image
Examples
Embodiment Construction
[0026] Such as figure 2 As shown, the schematic diagram of the network structure proposed by the present invention, in the structure, the first step is an image input layer, and the input image can be a single-channel grayscale image, or a 3-channel (RGB) color image. The size of the image can theoretically be any size, try to use the size of 32x32, 64x64, 224x224 and so on.
[0027] According to the above step 1, the second step is to perform convolution processing on the image. The size of all convolution kernels used in the present invention is set to 3×3 size, and the convolution layer contains multiple convolution operators acting on the input layer. On the image, the specific steps are as follows: W T X, where the X matrix is represented as multiple input images, where each column stores the pixel information of an image, the matrix W represents a convolution operator, each row represents a convolution operator, and the multiplication of two matrices is obtained. Th...
PUM
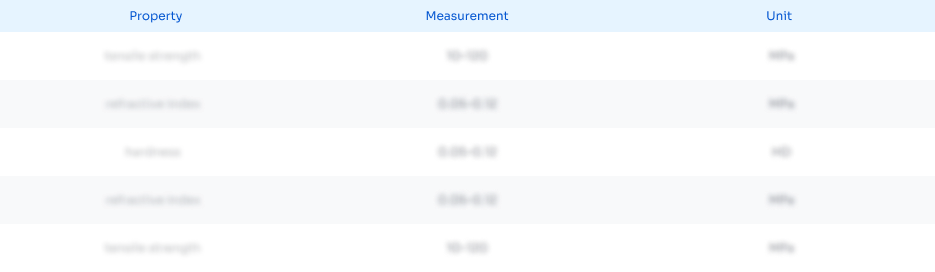
Abstract
Description
Claims
Application Information
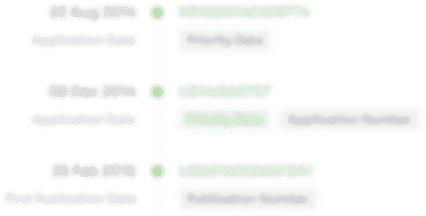
- R&D
- Intellectual Property
- Life Sciences
- Materials
- Tech Scout
- Unparalleled Data Quality
- Higher Quality Content
- 60% Fewer Hallucinations
Browse by: Latest US Patents, China's latest patents, Technical Efficacy Thesaurus, Application Domain, Technology Topic, Popular Technical Reports.
© 2025 PatSnap. All rights reserved.Legal|Privacy policy|Modern Slavery Act Transparency Statement|Sitemap|About US| Contact US: help@patsnap.com