High-order interactive multi-model filtering method based on mixture transition distribution
An interactive multi-model and model technology, applied in complex mathematical operations and other directions, can solve problems such as low precision, cumbersome setting process, and high-order Markov chain setting parameters, etc., to achieve high precision, solve setting difficulties, and reduce the possibility of sexual effect
- Summary
- Abstract
- Description
- Claims
- Application Information
AI Technical Summary
Problems solved by technology
Method used
Image
Examples
specific Embodiment approach 1
[0021] Embodiment 1: A high-order interactive multi-model filtering method based on mixed transfer distribution includes the following steps:
[0022] Step 1: Use the mixed transition distribution model to obtain the n-order model sequence transition probability ρ(m k |m k-n ,...,m k-1 );
[0023] Step 2: The estimated state vector is and the corresponding covariance is Perform real-time processing on time k; when k=1, go to step three; when k=2, go to step four; when 3≤k≤n, go to step six; when k>n, go to step Step seven;
[0024] Step 3: After initializing the state when k=1, turn to step 2 and wait for processing the radar observation data at the next k=k+1 moment;
[0025] Step 4: After initializing the state when k=2, go to Step 5;
[0026] Step 5: judge k, when k=n, the n-order model sequence probability U at time k k (m k-n+1 ,...,m k ), the estimated value of the n-order model sequence state and with corresponding covariance After initialization, turn...
specific Embodiment approach 2
[0029] Specific embodiment two: the difference between this embodiment and specific embodiment one is: adopt mixed transfer distribution model to obtain n-order model sequence transition probability ρ(m k | m k-n ,...,m k-1 ) The specific process is:
[0030]
[0031] where m j is the model at time j, j=k-n,...,k, if the number of models is r, then m j The value range of is from 1 to r; is from the model m k-g transfer to model m k The probability, is an element in the first-order Markov chain, λ g is each step factor, satisfying the following conditions:
[0032]
[0033] Other steps and parameters are the same as those in Embodiment 1.
specific Embodiment approach 3
[0034] Specific implementation mode three: the difference between this implementation mode and specific implementation mode one or two is: the described model is specifically:
[0035] x k+1 =F k (m k )X k +G k (m k )u k (m k )+Γ k (m k )v k (m k )
[0036] where X k is the x-axis position x at time k k , x-axis speed y-axis position y k , y-axis speed Composed of state vectors. f k (m k ) means that at time k the model m k The system transition matrix under, G k (m k ) is the input control matrix, u k (m k ) is the signal input, Γ k (m k ) is the noise coefficient matrix, v k (m k ) is the k-time model m k Zero-mean white Gaussian process noise under , with covariance Q k (m k ).
[0037]
[0038] Among them, T represents the sampling interval.
[0039] (1) When the model is a uniform motion model
[0040]
[0041] (2) When the model cooperates with the turning model
[0042]
[0043] (3) When the model uniformly accelerates the moti...
PUM
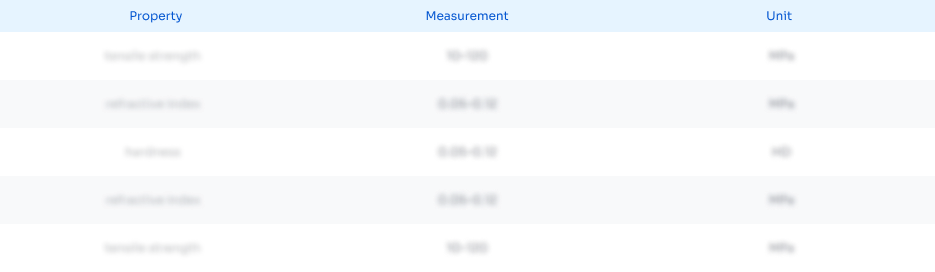
Abstract
Description
Claims
Application Information
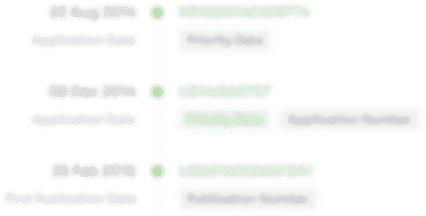
- R&D Engineer
- R&D Manager
- IP Professional
- Industry Leading Data Capabilities
- Powerful AI technology
- Patent DNA Extraction
Browse by: Latest US Patents, China's latest patents, Technical Efficacy Thesaurus, Application Domain, Technology Topic, Popular Technical Reports.
© 2024 PatSnap. All rights reserved.Legal|Privacy policy|Modern Slavery Act Transparency Statement|Sitemap|About US| Contact US: help@patsnap.com