Shift learning method and device for medical images
A technology of medical image and conversion method, which is applied in the field of medical image conversion learning, can solve the problems of consuming a lot of manpower and material resources, limited data volume, limited number of samples, etc., and achieve the effect of reducing difficulty and cost, reducing cost and conditions
- Summary
- Abstract
- Description
- Claims
- Application Information
AI Technical Summary
Problems solved by technology
Method used
Image
Examples
no. 1 example
[0068] The first embodiment: transforming and learning the parameters of the intelligent analysis chest X-ray model to the intelligent analysis chest CT model.
[0069] It is assumed that a model dedicated to analyzing chest X-rays has been acquired through the study and analysis of a large amount of data. Assuming that the data image dimension of each X-ray is (1, 4096, 4096), the dimension list of the model parameter matrix used is: [32, 3, 3, 3], [64, 32, 3, 3], [128, 64, 3, 3], [2, 4096].
[0070] At this time, you want to analyze the chest CT model. Assume that the dimension of each sub-image of chest CT is (300, 256, 256), and the list of model parameters used is: [32, 300, 3, 3], [64, 32 , 3, 3], [128, 64, 3, 3], [1000, 4096].
[0071] The chest X-ray model has sufficient data and sufficient training, so the model effect is very good, but the target training chest CT model has insufficient data, and the model is large and complex, and the training effect is very poor....
no. 2 example
[0078] The second embodiment: converting and learning the parameters of the intelligent analysis femoral head X-ray model to the intelligent analysis cardiopulmonary X-ray model.
[0079]It is assumed that an X-ray model dedicated to analyzing the femoral head has been obtained through the study and analysis of a large amount of data. Assuming that the data image dimension of each X-ray is (1, 2000, 2000), the dimension list of the model parameter matrix used is: [32, 3, 3, 3], [64, 32, 3, 3], [128, 64, 3, 3], [2, 1024].
[0080] At this time, I want to analyze the model, assuming that the dimension of each sub-image of cardiopulmonary X-ray is (1, 2000, 2000), the list of model parameters used is: [32, 3, 3, 3], [64, 32, 3, 3], [128, 64, 3, 3], [2, 1024].
[0081] The femoral head X-ray model has sufficient data and training, so the model effect is very good, but the target training cardiopulmonary X-ray model has insufficient data and the training effect is relatively poor...
no. 3 example
[0089] The third embodiment: transforming and learning the parameters of the intelligent analysis lung CT model to the intelligent analysis brain MRI model.
[0090] It is assumed that through the study and analysis of a large amount of data, there is a CT model specially used for analyzing lungs. Assuming that the input data image dimensions of the CT model are (1, 100, 512, 512), the dimension list of the model parameter matrix used is: [32, 100, 3, 3], [64, 32, 3, 3], [128, 64, 3, 3], [1, 1024].
[0091] At this time, I hope to analyze the model, assuming that the dimension of each sub-image of the brain MRI is (1, 100, 256, 256), and the list of model parameters used is: [32, 100, 3, 3], [64, 32 , 3, 3], [128, 64, 3, 3], [256, 128, 3, 3], [1, 1024].
[0092] The lung CT model has sufficient data and sufficient training, so the model effect is very good, but the target training brain MRI model has insufficient data, and the training effect is relatively poor. Therefore c...
PUM
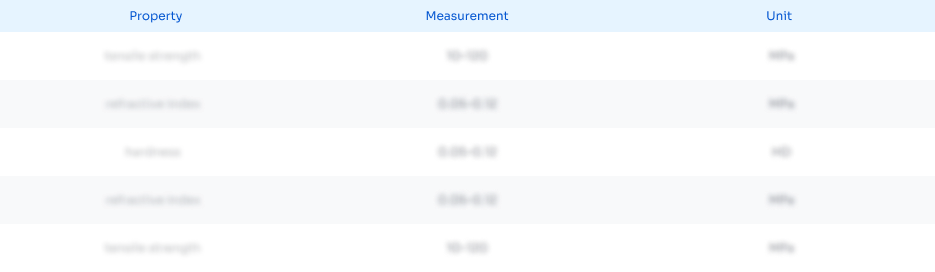
Abstract
Description
Claims
Application Information
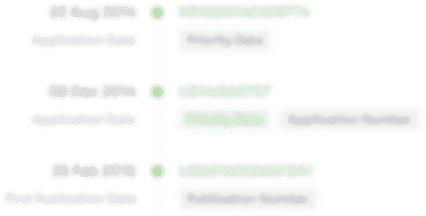
- R&D
- Intellectual Property
- Life Sciences
- Materials
- Tech Scout
- Unparalleled Data Quality
- Higher Quality Content
- 60% Fewer Hallucinations
Browse by: Latest US Patents, China's latest patents, Technical Efficacy Thesaurus, Application Domain, Technology Topic, Popular Technical Reports.
© 2025 PatSnap. All rights reserved.Legal|Privacy policy|Modern Slavery Act Transparency Statement|Sitemap|About US| Contact US: help@patsnap.com