Improved parallel channel convolutional neural network training method
A technology of convolutional neural network and training method, applied in the direction of neural learning method, biological neural network model, neural architecture, etc., can solve problems such as time-consuming and labor-intensive, network accuracy impact, network performance impact, etc., to overcome gradient instability , the effect of ensuring circulation
- Summary
- Abstract
- Description
- Claims
- Application Information
AI Technical Summary
Problems solved by technology
Method used
Image
Examples
Embodiment Construction
[0028] The improved parallel channel convolutional neural network training method provided by the present invention will be described in detail below in conjunction with the accompanying drawings and specific embodiments.
[0029] Such as figure 1 As shown, the improved parallel channel convolutional neural network training method provided by the invention comprises the following steps carried out in order:
[0030] 1) Use two parallel channels of direct connection and convolution to extract features from the data in the convolutional neural network, and obtain the feature matrix of the direct connection channel and the feature matrix of the convolution channel;
[0031] The data set composed of tens of thousands of color images with a size of 32×32 is input into the convolutional neural network. The present invention uses the CIFAR-10 data set composed of 60,000 color images with a size of 32×32, and then uses The two parallel channels of direct connection and convolution pe...
PUM
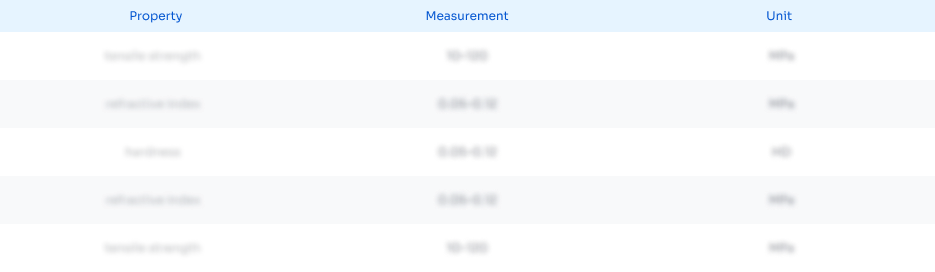
Abstract
Description
Claims
Application Information
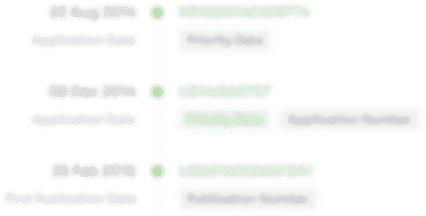
- R&D Engineer
- R&D Manager
- IP Professional
- Industry Leading Data Capabilities
- Powerful AI technology
- Patent DNA Extraction
Browse by: Latest US Patents, China's latest patents, Technical Efficacy Thesaurus, Application Domain, Technology Topic, Popular Technical Reports.
© 2024 PatSnap. All rights reserved.Legal|Privacy policy|Modern Slavery Act Transparency Statement|Sitemap|About US| Contact US: help@patsnap.com