DCNN (Deep Convolutional Neural Network)-DNN (Deep Neural Network) and PV-SVM (Paragraph Vector-Support Vector Machine)-based multi-modal depressive disorder estimation and classification method
A multi-modal, depression technology, applied in the field of computer and medicine, can solve the problems of time series information discarding, information not being mined, and the influence of feature dimension, so as to improve the detection accuracy, avoid over-fitting phenomenon, and the model is effective Effect
- Summary
- Abstract
- Description
- Claims
- Application Information
AI Technical Summary
Problems solved by technology
Method used
Image
Examples
Embodiment Construction
[0041] Concrete steps of the present invention are as follows:
[0042] Step 1, preprocessing the audio and video features by using the displacement range histogram and the Opensmile tool. This step is divided into two parts: (1) Input the video Landmarks feature into the displacement range histogram statistical method to obtain the global feature of the video Landmarks feature; (2) Input the audio LLD feature into the Opensmile tool to extract audio global statistics.
[0043] The steps of the displacement range histogram statistical method are as follows:
[0044] First define the time interval M:={M 1 , M 2 , M 3 ,...,M x}, range R := {R 1 , R 2 , R 3 ,...,R z}, the video Landmarks feature is used as the input of the displacement range histogram statistical method, and x and z represent the number of time intervals and ranges, respectively.
[0045] Then, for each time interval M x , calculate the Landmarks feature in each dimension, i+M x The difference between ...
PUM
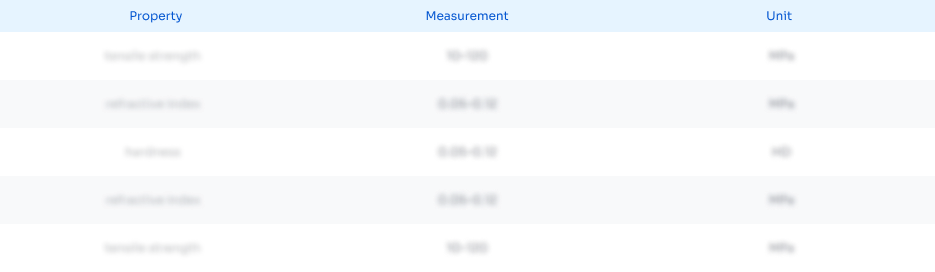
Abstract
Description
Claims
Application Information
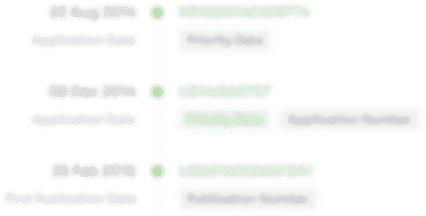
- R&D Engineer
- R&D Manager
- IP Professional
- Industry Leading Data Capabilities
- Powerful AI technology
- Patent DNA Extraction
Browse by: Latest US Patents, China's latest patents, Technical Efficacy Thesaurus, Application Domain, Technology Topic, Popular Technical Reports.
© 2024 PatSnap. All rights reserved.Legal|Privacy policy|Modern Slavery Act Transparency Statement|Sitemap|About US| Contact US: help@patsnap.com