Facial expression recognition method based on feature block weighting
A facial expression and recognition method technology, applied in the field of human facial expression recognition, can solve the problems of different contribution rates of facial expression recognition, and achieve the effect of improving recognition accuracy and improving commonality
- Summary
- Abstract
- Description
- Claims
- Application Information
AI Technical Summary
Problems solved by technology
Method used
Image
Examples
Embodiment 1
[0025] see figure 1 , the facial expression recognition method based on feature block weighting is characterized in that the operation steps are as follows: 1) extract the Gabor texture feature and geometric feature of the expression picture; 2) use the PCA algorithm to reduce the feature dimension for the extracted Gabor texture feature, and extract The geometric features are aligned in blocks, and the geometric features are divided into three feature blocks of the mouth, left eye, and right eye, and the Procrustes Analysis method is used to align each geometric feature; 3) Gabor texture features after PCA dimensionality reduction and The three geometric feature blocks after Procrustes Analysis are fused to form a fused feature; 4) The fused feature is input to the Bp neural network weighted by the feature block, and the neural network is trained to find the appropriate weight coefficient of each layer.
Embodiment 2
[0027] This embodiment is basically the same as Embodiment 1, and the special features are as follows:
[0028] The Gabor texture and the geometric feature of the described extraction expression picture are: adopt the Gabor filter to extract the Gabor texture feature of the expression image, adopt the Face++ function library to extract the geometric feature of the expression image.
[0029] The alignment of the geometric feature blocks is: divide the geometric features into left eye geometric feature blocks, right eye geometric feature blocks and mouth geometric feature blocks, and then use Procrustes Analysis to perform alignment processing on each feature block.
[0030] The feature fusion is: arranging and combining the Gabor feature and each geometric feature block in the form of a column vector.
[0031] The Bp neural network method of described characteristic block weighting is: before the input layer of neural network, increased weight layer, weight layer comprises four...
Embodiment 3
[0033] Such as figure 1 , using the Gabor filter to extract the Gabor features of facial expressions. Since the Gabor features have many feature dimensions, in high-dimensional feature representations, these features are usually linearly correlated and contain more useless or less useful variables, so , using the PCA algorithm to perform feature selection on the extracted Gabor features. Use the Face++ function library to extract the facial geometric features of the expression image. Due to the difference in the structure and size of the face, the position and size of the eyes and mouth are different, the extracted facial expression geometric features are divided into mouth, left eye, and right eye geometric features. block, and then perform Procrustes Analysis on each feature block of facial features separately. The extracted Gabor features are fused with the features after the block alignment of the geometric features to form the fused features. The fusion method is as foll...
PUM
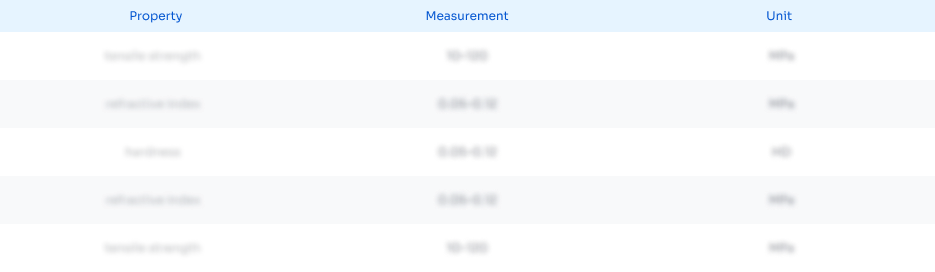
Abstract
Description
Claims
Application Information
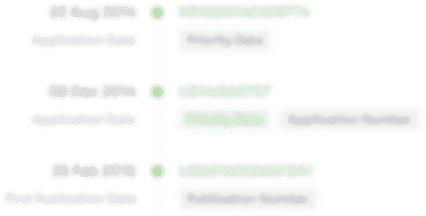
- R&D
- Intellectual Property
- Life Sciences
- Materials
- Tech Scout
- Unparalleled Data Quality
- Higher Quality Content
- 60% Fewer Hallucinations
Browse by: Latest US Patents, China's latest patents, Technical Efficacy Thesaurus, Application Domain, Technology Topic, Popular Technical Reports.
© 2025 PatSnap. All rights reserved.Legal|Privacy policy|Modern Slavery Act Transparency Statement|Sitemap|About US| Contact US: help@patsnap.com