Wind turbine active power prediction and error correction method based on neural network
A technology for active power and error correction, applied in the field of wind energy forecasting, which can solve problems such as difficulty in solving, high cost, and forecasting error.
- Summary
- Abstract
- Description
- Claims
- Application Information
AI Technical Summary
Problems solved by technology
Method used
Image
Examples
Embodiment Construction
[0075] In order to make the object, technical solution and advantages of the present invention more clear, the present invention will be further described in detail below in conjunction with the examples. It should be understood that the specific embodiments described here are only used to explain the present invention, not to limit the present invention.
[0076] The application principle of the present invention will be described in detail below in conjunction with the accompanying drawings.
[0077] like figure 1 As shown, a neural network-based wind turbine active power prediction and error correction method includes the following steps:
[0078] S1: Read in the original sampling active power time series p={p(i),i=1,2,...,N} of the wind turbine, where N is the number of sampling points of the original active power of the wind turbine; adjust p is the average active power time series p'={p'(j),j=1,2,...,M} according to the forecast interval requirements, where M is the av...
PUM
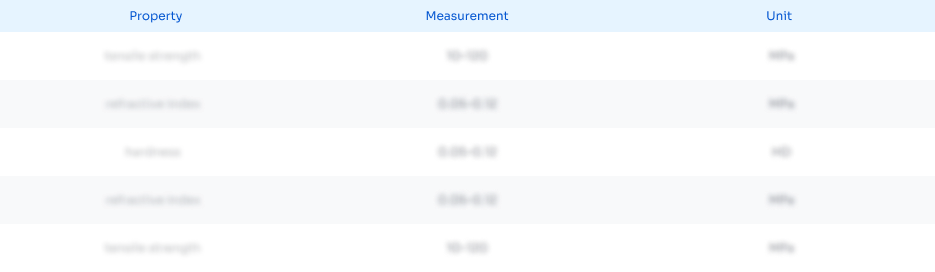
Abstract
Description
Claims
Application Information
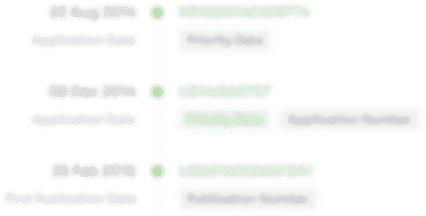
- R&D
- Intellectual Property
- Life Sciences
- Materials
- Tech Scout
- Unparalleled Data Quality
- Higher Quality Content
- 60% Fewer Hallucinations
Browse by: Latest US Patents, China's latest patents, Technical Efficacy Thesaurus, Application Domain, Technology Topic, Popular Technical Reports.
© 2025 PatSnap. All rights reserved.Legal|Privacy policy|Modern Slavery Act Transparency Statement|Sitemap|About US| Contact US: help@patsnap.com