Short-period power combination prediction method for variable-weight-coefficient grid-connected photovoltaic power station
A technology for weight coefficients and photovoltaic power plants, applied in data processing applications, instruments, resources, etc., can solve problems such as difficulty in prediction accuracy, uncertainty, and difficult meteorological factor analysis, so as to reduce the probability of extreme errors and make up for it. The effect of large prediction error and simplified complexity
- Summary
- Abstract
- Description
- Claims
- Application Information
AI Technical Summary
Problems solved by technology
Method used
Image
Examples
Embodiment Construction
[0042] The present invention will be described in detail below in conjunction with the accompanying drawings and specific embodiments. This embodiment is carried out on the premise of the technical solution of the present invention, and detailed implementation and specific operation process are given, but the protection scope of the present invention is not limited to the following embodiments.
[0043]Based on the established single prediction model and equal weight prediction model, this method proposes a combination prediction model with variable weight coefficients to realize the prediction of the output power of photovoltaic power plants, and can adjust the weight coefficients of multiple single prediction models in real time . The proposed method combines gray relational analysis with BP neural network to obtain a variable weight coefficient combination model, and realizes the use of support vector machine model (SVM), gray relational analysis combined with support vecto...
PUM
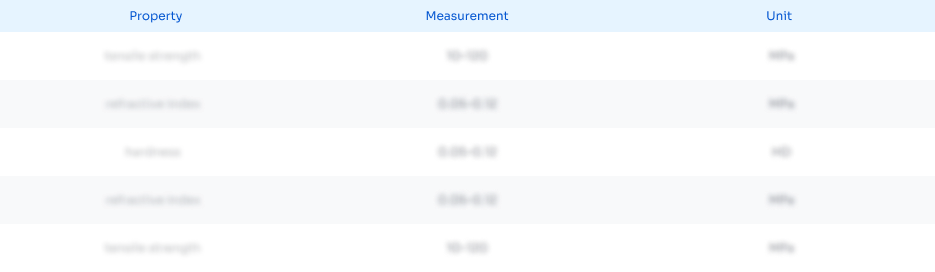
Abstract
Description
Claims
Application Information
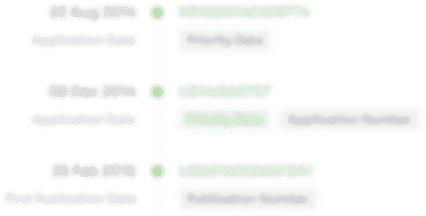
- R&D Engineer
- R&D Manager
- IP Professional
- Industry Leading Data Capabilities
- Powerful AI technology
- Patent DNA Extraction
Browse by: Latest US Patents, China's latest patents, Technical Efficacy Thesaurus, Application Domain, Technology Topic, Popular Technical Reports.
© 2024 PatSnap. All rights reserved.Legal|Privacy policy|Modern Slavery Act Transparency Statement|Sitemap|About US| Contact US: help@patsnap.com