Imbalanced data industrial fault classification method based on k-means
A fault classification and fault class technology, applied in the direction of instruments, character and pattern recognition, computer parts, etc., can solve the problems of overfitting, adding system methods, and the application effect is not very ideal, so as to solve the problem of unbalanced data classification. problems, increase classification accuracy, reduce the effect of overfitting
- Summary
- Abstract
- Description
- Claims
- Application Information
AI Technical Summary
Problems solved by technology
Method used
Image
Examples
Embodiment Construction
[0013] The present invention is aimed at the problem of fault classification in industrial processes. The method first uses k-means, and clusters the classes with more data according to the degree of imbalance, divides the majority class into N subclasses, and then combines them with M minority classes , as a multi-classification problem of (M+N) class, and finally learn according to the Naive Bayesian classifier.
[0014] The main steps of the technical solution adopted in the present invention are respectively as follows:
[0015] Step 1: Use the system to collect the data of the normal working conditions of the process and various fault data to form a labeled training sample set for modeling: Assume that the fault category is C, add a normal class, and the total modeling data of each sample The category is C+1, ie i=1,2...C+1 where no i is the number of training samples, m is the number of process variables, and R is the set of real numbers. So the complete labeled tr...
PUM
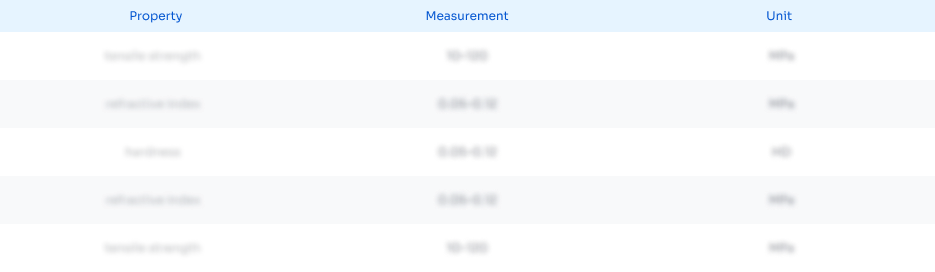
Abstract
Description
Claims
Application Information
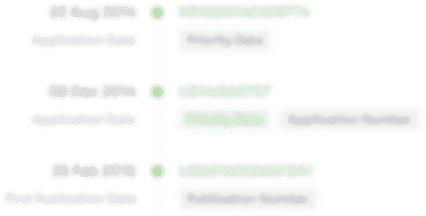
- R&D Engineer
- R&D Manager
- IP Professional
- Industry Leading Data Capabilities
- Powerful AI technology
- Patent DNA Extraction
Browse by: Latest US Patents, China's latest patents, Technical Efficacy Thesaurus, Application Domain, Technology Topic, Popular Technical Reports.
© 2024 PatSnap. All rights reserved.Legal|Privacy policy|Modern Slavery Act Transparency Statement|Sitemap|About US| Contact US: help@patsnap.com