Speaker recognition method based on three-dimensional convolutional neural network text independence and system
A technology of speaker recognition and neural network, which is applied in the field of text-independent speaker recognition and speaker recognition, can solve the problems of reducing experience, cumbersome steps, and heavy workload, and achieves the effect of improving differentiation and improving experience.
- Summary
- Abstract
- Description
- Claims
- Application Information
AI Technical Summary
Problems solved by technology
Method used
Image
Examples
Embodiment 1
[0068] Take training a model containing 1000 speakers as an example to illustrate the speaker recognition model training process.
[0069] (1) Collect samples of each speaker, indicator: number of samples 3000 samples per person;
[0070] (2) The voice preprocessing module processes all voice data to obtain three-dimensional training data;
[0071] (3) Divide all training data into 4:1 randomly and use them as training set and validation set;
[0072] (4) The residual network training model is used, and the model training is terminated when the recognition accuracy of the model on the verification set remains basically unchanged, and an offline speaker recognition model is obtained.
[0073] Registration mode
[0074] (1) Voice sample collection
[0075] Collect training samples by recording;
[0076] (2) Voice preprocessing
[0077] Use the voice preprocessing module to preprocess the voice to generate registration data;
[0078] (3) Feature extraction
[0079] The offline model generated in...
Embodiment 2
[0081] Take the registration of a data set containing 10 speakers as an example to illustrate the speaker registration process.
[0082] (1) Collect the voice data of 10 speakers, with 20 voice data samples per person;
[0083] (2) The voice preprocessing module processes all voice data to obtain three-dimensional data of each speaker;
[0084] (3) Use the offline model generated in the training phase to extract features, and save the features of each person in the database, speaker0, speaker1,..., speaker9;
[0085] Recognition mode
[0086] (1) Voice sample collection
[0087] Collect training samples by recording.
[0088] (2) Voice preprocessing
[0089] The offline model generated in the training phase is used to extract features from the preprocessed speech to generate test data.
[0090] (3) Extract features
[0091] The offline model generated in the training phase is used to extract features of the preprocessed speech.
[0092] (4) Feature comparison
[0093] Find the cosine distance ...
Embodiment 3
[0095] Take identifying a speaker as an example to illustrate the process of speaker identification.
[0096] (1) Collect one piece of voice data of the speaker;
[0097] (2) The voice preprocessing module processes all voice data, and duplicates the test sample repeatedly according to the depth of the three-dimensional data used in the training data to obtain the three-dimensional data of this sample;
[0098] (3) The offline model generated in the training phase is used to extract features;
[0099] (4) This feature and the feature registered in the database have cosine distances to get sim0, sim1,..., sim9, find the maximum value sim_max of these 10 similarities and the speaker_x of the corresponding speaker, if the maximum value is greater than Threshold sim, the sample is accepted as speaker_x, otherwise, it is recognized as an unregistered speaker.
[0100] In summary, the present invention implements a text-independent speaker recognition method and system based on a three-dimen...
PUM
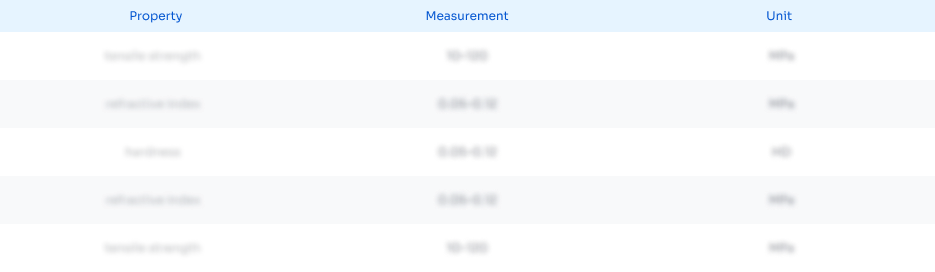
Abstract
Description
Claims
Application Information
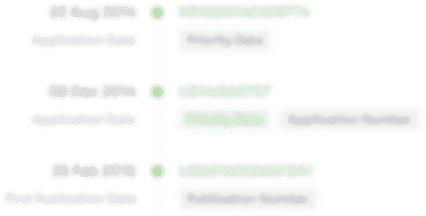
- R&D Engineer
- R&D Manager
- IP Professional
- Industry Leading Data Capabilities
- Powerful AI technology
- Patent DNA Extraction
Browse by: Latest US Patents, China's latest patents, Technical Efficacy Thesaurus, Application Domain, Technology Topic, Popular Technical Reports.
© 2024 PatSnap. All rights reserved.Legal|Privacy policy|Modern Slavery Act Transparency Statement|Sitemap|About US| Contact US: help@patsnap.com