Convolutional neural network-based road adhesion coefficient identification method
A convolutional neural network and road adhesion coefficient technology, applied in the field of image recognition road surface, can solve the problems of not considering lateral dynamics, not suitable for the estimation of road adhesion coefficient, estimation error, etc.
- Summary
- Abstract
- Description
- Claims
- Application Information
AI Technical Summary
Problems solved by technology
Method used
Image
Examples
Embodiment Construction
[0057] The technical solutions in the embodiments of the present invention will be described clearly and in detail below with reference to the drawings in the embodiments of the present invention. The described embodiments are only some of the embodiments of the invention.
[0058] The technical scheme that the present invention solves the problems of the technologies described above is:
[0059] A road surface adhesion coefficient identification method based on a convolutional neural network according to the present invention includes the collection of pictures of different road surface conditions, the calibration of road surface adhesion coefficients, and the establishment of a database of pictures of various road surface conditions. Use the image segmentation algorithm to judge the position marked by the characteristic area of each road condition picture in the database, and extract the sensitive area of different pixel sizes; input it as a training sample into the conv...
PUM
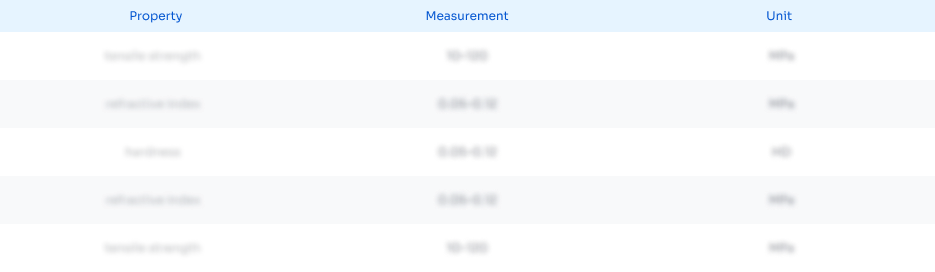
Abstract
Description
Claims
Application Information
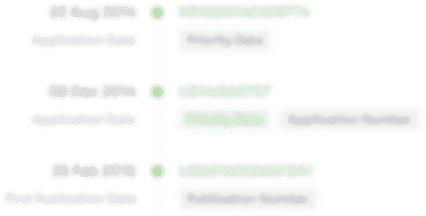
- R&D Engineer
- R&D Manager
- IP Professional
- Industry Leading Data Capabilities
- Powerful AI technology
- Patent DNA Extraction
Browse by: Latest US Patents, China's latest patents, Technical Efficacy Thesaurus, Application Domain, Technology Topic, Popular Technical Reports.
© 2024 PatSnap. All rights reserved.Legal|Privacy policy|Modern Slavery Act Transparency Statement|Sitemap|About US| Contact US: help@patsnap.com