Multiple-model self-calibration Kalman filter (MSKF) method
A Kalman filtering and self-calibration technology, applied in the field of robust Kalman filtering, which can solve problems such as poor filtering accuracy
- Summary
- Abstract
- Description
- Claims
- Application Information
AI Technical Summary
Problems solved by technology
Method used
Image
Examples
Embodiment Construction
[0097] The present invention will be described in detail below in conjunction with the accompanying drawings.
[0098] The present invention proposes a multi-model self-calibration Kalman filtering method, the flow chart of which is as follows figure 1 As shown, the time update flow chart is as follows figure 2 As shown, it includes the following six steps:
[0099] Step 1: Establish the basic equations of the system
[0100]
[0101]
[0102] Z k =H k x k +V k (34)
[0103] In the formula, X k represents the state vector of the system, and Corresponding to the kinetic model with unknown input and the standard kinetic model, Z k Indicates the system measurement vector, Φ k and H k are state transition matrix and measurement matrix, respectively, b k Indicates unknown input, W k with V k are the system noise vector and the measurement noise vector respectively, and their variance matrices are Q k and R k , and satisfy
[0104]
[0105] In the form...
PUM
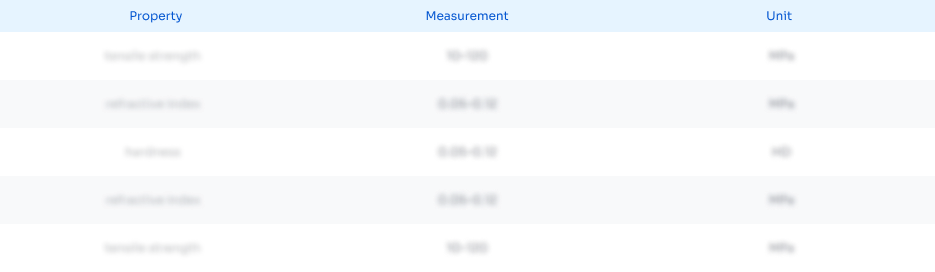
Abstract
Description
Claims
Application Information
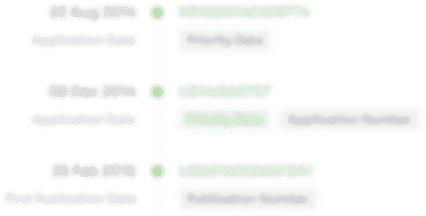
- R&D
- Intellectual Property
- Life Sciences
- Materials
- Tech Scout
- Unparalleled Data Quality
- Higher Quality Content
- 60% Fewer Hallucinations
Browse by: Latest US Patents, China's latest patents, Technical Efficacy Thesaurus, Application Domain, Technology Topic, Popular Technical Reports.
© 2025 PatSnap. All rights reserved.Legal|Privacy policy|Modern Slavery Act Transparency Statement|Sitemap|About US| Contact US: help@patsnap.com