A depression auxiliary detection method based on acoustic characteristics and sparse mathematics and a classifier
An acoustic feature and auxiliary detection technology, applied in instruments, speech analysis, psychological devices, etc., can solve the problems of lack of objective evaluation indicators, high misjudgment rate, single detection and screening methods, etc.
- Summary
- Abstract
- Description
- Claims
- Application Information
AI Technical Summary
Problems solved by technology
Method used
Image
Examples
Embodiment 1
[0084] The working conditions of the depression speech recognition system need to provide a quiet environment. Once background noise is introduced, the performance of the recognition system will be affected. Therefore, this embodiment provides a method for enhancing speech quality based on improved spectral subtraction, which specifically includes the following steps :
[0085] Step 1: Assuming that speech is a stationary signal, while noise and speech are additive signals and are not correlated with each other, the noisy speech signal can be expressed as:
[0086] y(n)=s(n)+d(n), 0≤n≤N-1 (1)
[0087] Where s(n) is a pure speech signal, d(n) is a stationary additive Gaussian noise, and y(n) is a noisy speech signal. Represent the noisy speech signal in the frequency domain, where * represents the complex conjugate, so:
[0088] |Y k | 2 =|S k | 2 +|N k | 2 +S k N k * +S k * N k (2)
[0089] Step 2: Assume that the noise is uncorrelated, that is, s(n) and d(n) a...
Embodiment 2
[0100] The embodiment of the present invention extracts the characteristic parameters (fundamental frequency, formant, energy, and short-term average amplitude) of different emotional voices based on the signal enhancement in the first embodiment. Five kinds of statistical feature parameters (maximum value, minimum value, variation range, mean value, variance) are used to record commonly used emotion recognition, so as to reflect the voice characteristics of depressed patients and the differences from the other two types of emotional voices, specifically including the following steps:
[0101] Step 1: Read in the voice data and preprocess it. After detecting the endpoint of the voice data, take out a frame of voice data and add a window, calculate the cepstrum, and then look for the peak near the expected pitch period. If the peak value of the cepstrum exceeds the expected setting If the threshold is determined, the input speech segment is defined as voiced, and the position of...
Embodiment 3
[0116] In the embodiment of the present invention, based on speech recognition and facial emotion recognition, an auxiliary judgment is made on whether suffering from depression, which specifically includes the following steps:
[0117] Step 1: Read in the voice data and preprocess it, and use the method in Embodiment 1 to perform signal enhancement on all voices.
[0118] Step 2: Select the standard 3-layer BP neural network to input the three types of voices of fear, normal and depression respectively in order, and extract 12 eigenvalues of MFCC to form a 12-dimensional feature vector. Therefore, the number of nodes in the input layer of the BP neural network is 12. The number of nodes in the output layer of the meta-network is determined by the number of categories, and the three speech emotions are recognized, so the number of nodes in the output layer of the BP neural network is 3, and the number of nodes in the hidden layer is 6. When training the network, if the input...
PUM
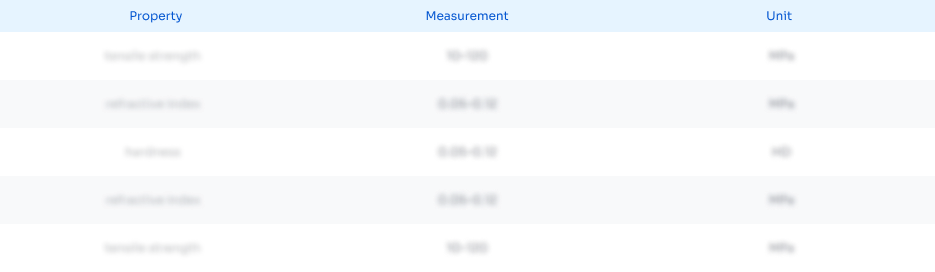
Abstract
Description
Claims
Application Information
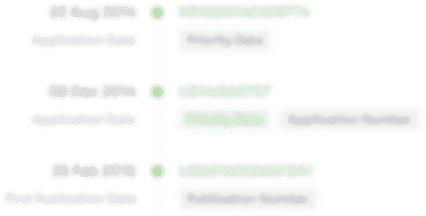
- R&D Engineer
- R&D Manager
- IP Professional
- Industry Leading Data Capabilities
- Powerful AI technology
- Patent DNA Extraction
Browse by: Latest US Patents, China's latest patents, Technical Efficacy Thesaurus, Application Domain, Technology Topic.
© 2024 PatSnap. All rights reserved.Legal|Privacy policy|Modern Slavery Act Transparency Statement|Sitemap