Super-resolution implementation method based on reconstruction optimization and deep neural network
A deep neural network and super-resolution technology, which is applied in the field of super-resolution realization based on reconstruction optimization and deep neural network, can solve problems such as image information inconsistency and errors, and achieve excellent performance.
- Summary
- Abstract
- Description
- Claims
- Application Information
AI Technical Summary
Problems solved by technology
Method used
Image
Examples
Embodiment Construction
[0029] The present invention will be described in detail below in conjunction with the accompanying drawings and specific embodiments.
[0030] The present invention first adopts a reconstruction-based super-resolution technique, taking multiple low-resolution image sequences with sub-pixel offsets as input. Considering the ill-conditioned nature of the problem, the L1 norm is added to the objective function to constrain the solution process. The L1 regularization term helps to generate a sparse weight matrix, which in turn can be used for feature selection. The objective function is quickly converged by conjugate gradients to obtain a high-resolution image. In reconstruction-based super-resolution techniques, ringing inevitably occurs when the magnification factor is too large or the input image is insufficient. In the present invention, a three-layer fully convolutional neural network is used to suppress the ringing phenomenon, and further optimize the previously output hi...
PUM
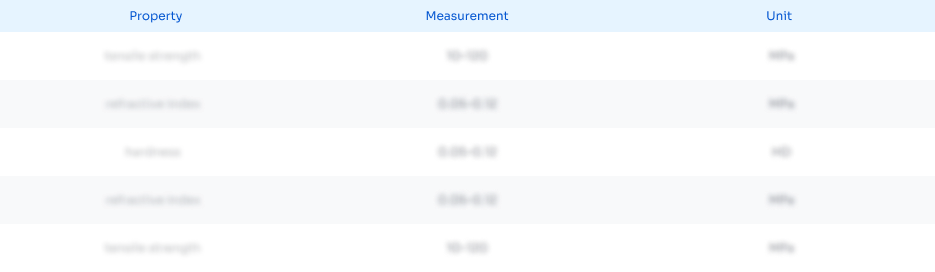
Abstract
Description
Claims
Application Information
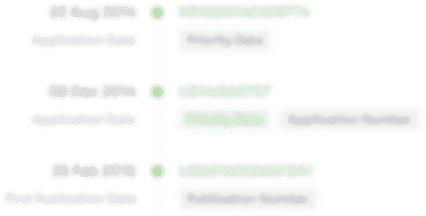
- R&D
- Intellectual Property
- Life Sciences
- Materials
- Tech Scout
- Unparalleled Data Quality
- Higher Quality Content
- 60% Fewer Hallucinations
Browse by: Latest US Patents, China's latest patents, Technical Efficacy Thesaurus, Application Domain, Technology Topic, Popular Technical Reports.
© 2025 PatSnap. All rights reserved.Legal|Privacy policy|Modern Slavery Act Transparency Statement|Sitemap|About US| Contact US: help@patsnap.com