Classification method for arrhythmia based on one-dimension convolution neural-network and S transformation
A convolutional neural network and arrhythmia technology, which is applied in the measurement of pulse rate/heart rate, medical science, sensors, etc., can solve the problems of large amount of calculation, ECG signal cannot express the relationship between time and frequency domain, and the classification effect is not obvious. , to achieve the effect of improving accuracy, speeding up convergence, and fast convergence
- Summary
- Abstract
- Description
- Claims
- Application Information
AI Technical Summary
Problems solved by technology
Method used
Image
Examples
specific example
[0072] The ECG signal data of this example comes from public databases. The specific process of the example is as follows:
[0073] (1) Preprocessing: In terms of noise removal, the median filter method is used to remove baseline drift, and the low-pass filter is used to remove power line interference and high-frequency noise. R wave detection uses amplitude threshold and wavelet threshold.
[0074] (2) Feature extraction: such as figure 2 As shown, according to the R position detected by the preprocessing, the 90 sampling point signals before the R wave position, the 197 sampling point signals after the R wave position, a total of 288 sampling points of the ECG segment, extract 1D-CNN features and time-frequency domain characteristics.
[0075] The 1D-CNN features of this example are: build a 3-layer 1D-CNN network, and each layer of CNN network contains a convolutional layer and a pooling layer. The size of the convolution kernel is 3, and the numbers are 64, 128, and 2...
PUM
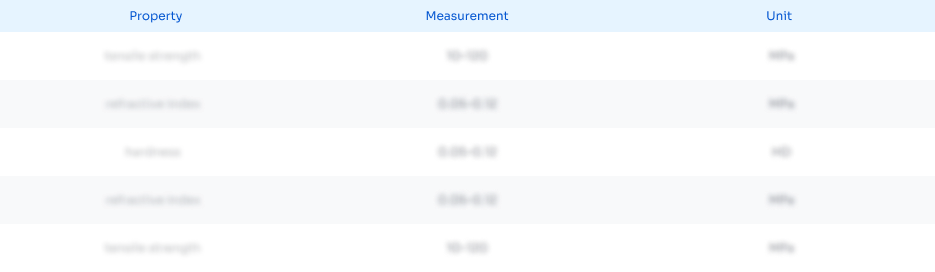
Abstract
Description
Claims
Application Information
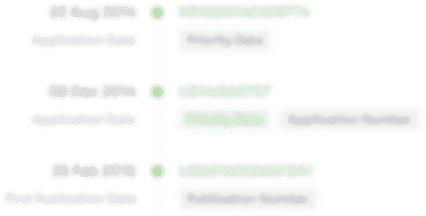
- R&D Engineer
- R&D Manager
- IP Professional
- Industry Leading Data Capabilities
- Powerful AI technology
- Patent DNA Extraction
Browse by: Latest US Patents, China's latest patents, Technical Efficacy Thesaurus, Application Domain, Technology Topic, Popular Technical Reports.
© 2024 PatSnap. All rights reserved.Legal|Privacy policy|Modern Slavery Act Transparency Statement|Sitemap|About US| Contact US: help@patsnap.com