Non-rigid multiscale object detection method based on convolutional neural network
A convolutional neural network and object detection technology, applied in the field of detection of non-rigid multi-scale objects, can solve the problems of low universality and robustness of detection methods, and achieve a simple framework, improved performance, and high portability Effect
- Summary
- Abstract
- Description
- Claims
- Application Information
AI Technical Summary
Problems solved by technology
Method used
Image
Examples
Embodiment Construction
[0023] The present invention will be further described below in conjunction with the accompanying drawings and specific embodiments.
[0024] Such as figure 1 As shown, the present invention is a non-rigid multi-scale object detection method based on convolutional neural network. The specific steps of the method are as follows:
[0025] Step 1. Annotated image;
[0026] figure 1 represents the entire training process. Input the labeled training data (image to be labeled) into the neural network, and obtain the output result through forward propagation. Compare the output result with the label to get the error (measured by the loss function, the goal of training is to reduce the loss function), update the parameters through the error gradient backpropagation of the neural network, and so on, so that the loss function decreases and converges.
[0027] In the training process, in addition to using the traditional translation, multi-scale, and image contrast adjustment metho...
PUM
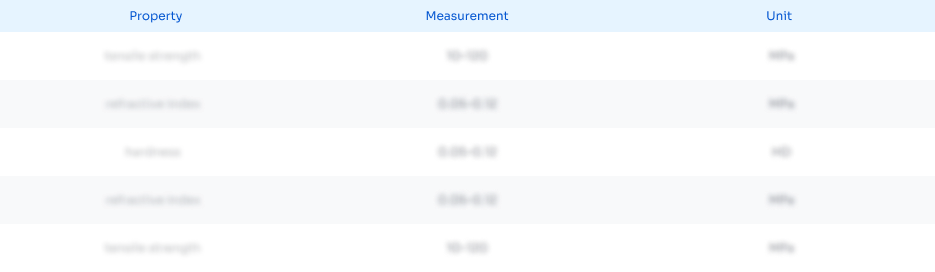
Abstract
Description
Claims
Application Information
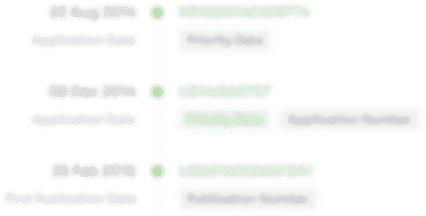
- R&D Engineer
- R&D Manager
- IP Professional
- Industry Leading Data Capabilities
- Powerful AI technology
- Patent DNA Extraction
Browse by: Latest US Patents, China's latest patents, Technical Efficacy Thesaurus, Application Domain, Technology Topic.
© 2024 PatSnap. All rights reserved.Legal|Privacy policy|Modern Slavery Act Transparency Statement|Sitemap