Multi-element time sequence prediction method
A multivariate time series and sequence forecasting technology, which is applied in forecasting, neural learning methods, biological neural network models, etc., can solve problems such as difficult to reflect the impact of historical loads, and cannot meet the needs of energy load forecasting, and achieve the effect of improving accuracy
- Summary
- Abstract
- Description
- Claims
- Application Information
AI Technical Summary
Problems solved by technology
Method used
Image
Examples
Embodiment 1
[0044] Reference figure 1 , figure 1 It shows a flowchart of a multivariate time series forecasting method provided by the present invention. Including: Step 1 to Step 4.
[0045] Step 1. Data preprocessing: seasonal and trend decomposition algorithm (STL-Seasonal and Trend decomposition using Loess) based on local regression (LOESS-LOcal regrESSion), which decomposes multivariate time series seasonally into trend series, cyclic series and irregular series .
[0046] Step two, trend sequence prediction: use linear or nonlinear regression algorithm to predict the trend sequence to obtain the trend sequence forecast value.
[0047] Step 3: Circulation sequence prediction: Based on the combined model, the cyclic sequence that introduces external factors and historical variables is predicted to obtain the initial prediction values of multiple cyclic sequences, and the initial prediction values are merged by the feedforward neural network to obtain the cyclic sequence prediction ...
PUM
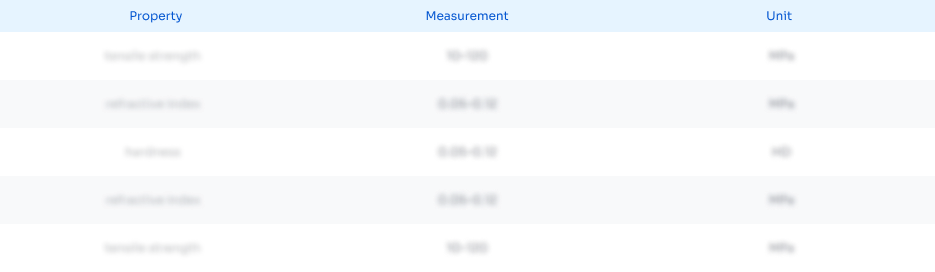
Abstract
Description
Claims
Application Information
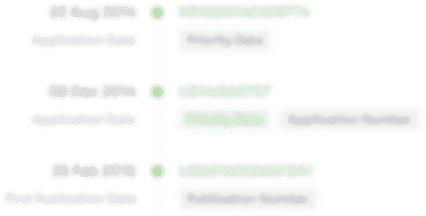
- R&D
- Intellectual Property
- Life Sciences
- Materials
- Tech Scout
- Unparalleled Data Quality
- Higher Quality Content
- 60% Fewer Hallucinations
Browse by: Latest US Patents, China's latest patents, Technical Efficacy Thesaurus, Application Domain, Technology Topic, Popular Technical Reports.
© 2025 PatSnap. All rights reserved.Legal|Privacy policy|Modern Slavery Act Transparency Statement|Sitemap|About US| Contact US: help@patsnap.com