Offshore oil-gas platform detection method based on structural recurrent neural network
A technology of recursive neural network and detection method, which is applied in the field of remote sensing image offshore oil and gas platform detection, can solve the problems of low target detection accuracy and large amount of calculation, and achieve the effect of strong feature capability, high detection rate accuracy and accurate detection
- Summary
- Abstract
- Description
- Claims
- Application Information
AI Technical Summary
Problems solved by technology
Method used
Image
Examples
Embodiment 1
[0052] Embodiment 1: The present invention will be further described below in conjunction with the accompanying drawings.
[0053] figure 1 It is a block diagram of the main structure of the method of the present invention. The detection method of the remote sensing image offshore platform based on the recursive structural neural network and the bidirectional circular convolution neural network mainly includes two parts: the first part, the high-order remote sensing imaging of the offshore platform based on the structural recurrent neural network spatiotemporal features. In the second part, a two-way cyclic convolutional neural network deep learning architecture is established to achieve accurate detection of offshore oil and gas platforms. The input of the first part is the original image, the output of the first part is used as the input of the second part, and the output of the second part is the detection result.
[0054] figure 2 is a high-order spatio-temporal charac...
Embodiment 2
[0058] Embodiment 2: The technical solution of the present invention and the scientific principles on which it is based are described in detail below.
[0059] 1. Structural Recursive Neural Network (S-RNN)
[0060] (1) Share factors between nodes
[0061] Starting with the time-space image, each factor in the structural recursive neural network model is represented by a recursive neural network (Recursive Neural Network, RNN). The RNN model is connected by a structure that can capture the time-space image structure and interconnection. Each factor in the space-time graph has parameters, and similar nodes can share factors and parameters without learning the characteristics of each factor. Assuming that all factor nodes in the space-time graph can share common node factors and parameters, this model can strengthen the sharing between similar nodes, and can further strengthen the processing of spatio-temporal images through nodes without increasing parameters. flexibility. M...
Embodiment 3
[0081] Embodiment 3: The method for detecting offshore oil and gas platforms in remote sensing images based on structural recursive neural network. The specific implementation steps are as follows:
[0082] Step 1, according to the serialized structural characteristics of spatio-temporal images, a structural recurrent neural network model of maritime targets is established;
[0083] Step 1.1, select a certain number of multi-temporal optical remote sensing images with different resolutions and multi-viewpoints that can be visually recognized by the oil and gas platform, and then obtain the image set A1;
[0084] Step 1.2, manually calibrate the image set A1 obtained in step 1.1, mark the platform target and interference target, and then obtain the marked remote sensing target image set A2;
[0085] Step 1.3, perform basic image preprocessing on the remote sensing image set A1, and obtain the target saliency image set A3;
[0086] Step 1.4, on the basis of the image set A3, ac...
PUM
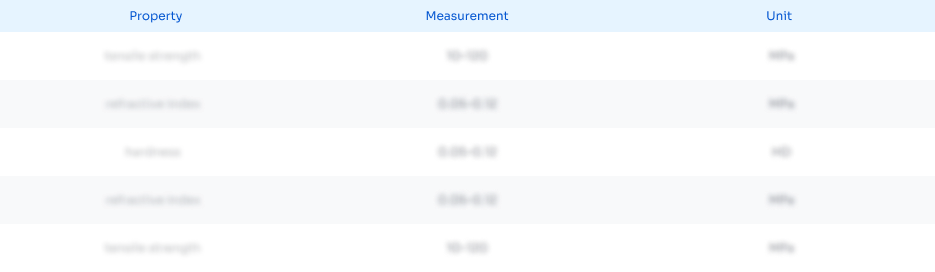
Abstract
Description
Claims
Application Information
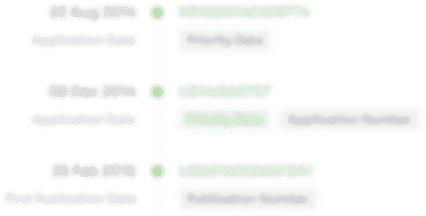
- R&D Engineer
- R&D Manager
- IP Professional
- Industry Leading Data Capabilities
- Powerful AI technology
- Patent DNA Extraction
Browse by: Latest US Patents, China's latest patents, Technical Efficacy Thesaurus, Application Domain, Technology Topic.
© 2024 PatSnap. All rights reserved.Legal|Privacy policy|Modern Slavery Act Transparency Statement|Sitemap