Deep learning recognition method for ferrite defects based on Fisher criterion
A technology of learning recognition and defect depth, which is applied in the field of surface defect recognition of rectangular ferrite magnetic sheets, can solve the problems of low efficiency and high error rate, and achieve the effect of strengthening detection speed, high accuracy and improving detection accuracy
- Summary
- Abstract
- Description
- Claims
- Application Information
AI Technical Summary
Problems solved by technology
Method used
Image
Examples
Embodiment Construction
[0057] The present invention will be further described below in conjunction with the accompanying drawings.
[0058] A deep learning recognition method for ferrite defects based on Fisher's criterion includes the following steps:
[0059] Step 1: Obtain images of rectangular ferrite magnetic sheets of known defects and defect-free samples through an industrial camera;
[0060] Step 2: Obtain images of defective and non-defective ferrite samples;
[0061] Step 3: Define the noise reduction and denoising autoencoder SDA;
[0062] The specific processing method is as follows:
[0063] (1) Define the vectors z and y respectively, and the calculation formula is as follows:
[0064] z=s(Wx+b)
[0065] y=g θ '(z)=s(W'z+b)
[0066] Among them, W is the weight matrix, b is the input bias, s() is the sigmoid function, z is a d'-dimensional vector, x is a d-dimensional input vector, y is also a d-dimensional vector as an approximate vector of x, and the x and y vector elements are ...
PUM
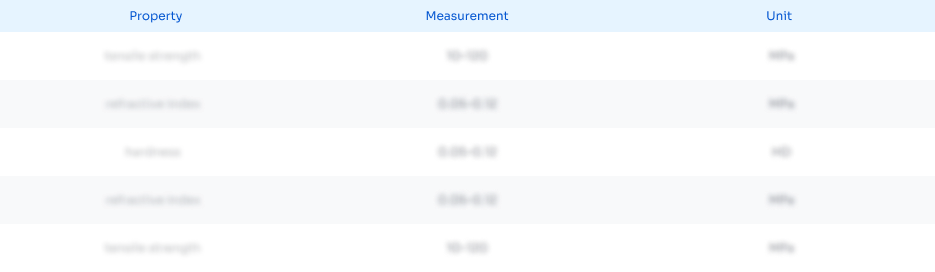
Abstract
Description
Claims
Application Information
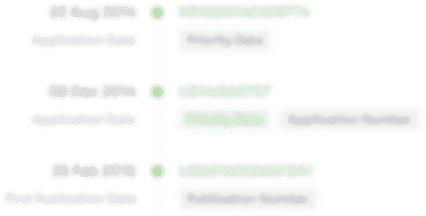
- R&D Engineer
- R&D Manager
- IP Professional
- Industry Leading Data Capabilities
- Powerful AI technology
- Patent DNA Extraction
Browse by: Latest US Patents, China's latest patents, Technical Efficacy Thesaurus, Application Domain, Technology Topic, Popular Technical Reports.
© 2024 PatSnap. All rights reserved.Legal|Privacy policy|Modern Slavery Act Transparency Statement|Sitemap|About US| Contact US: help@patsnap.com