Depth video significance detection method based on motion and memory information
A detection method and depth video technology, applied in the field of computer vision, can solve the problems of low-quality and dynamic video information, insufficient understanding of advanced semantic information, and inability to make full use of inter-frame information to achieve the effect of ensuring accuracy
- Summary
- Abstract
- Description
- Claims
- Application Information
AI Technical Summary
Problems solved by technology
Method used
Image
Examples
Embodiment 1
[0050]The embodiment of the present invention is based on the fully convolutional neural network, and the depth video eye positioning point detection technology that considers motion and memory information cooperatively, analyzes and fully understands the original video data, see figure 1 and figure 2 , its main process is divided into the following five parts:
[0051] 101: Obtain a detection data set consisting of an image salient object detection data set and a video eye positioning point detection data set; perform ground truth map calculation on the video eye positioning point detection data set, and obtain the final eye positioning map of the current frame ;
[0052] 102: Construct four models for extracting local information and global information with different deconvolution layers;
[0053] 103: Pre-train the four models on the image salient object detection dataset, and then perform model fine-tuning on the pre-trained four models on the video eye positioning poin...
Embodiment 2
[0076] The scheme in embodiment 1 is further introduced below in conjunction with specific calculation formulas, accompanying drawings, examples, and Table 1-Table 3, see the following description for details:
[0077] 201: Data set production;
[0078] In order to improve the generalization ability of the model, this method selects 8 most commonly used data sets for image saliency detection and video saliency detection to make a data set suitable for this task. Among them, there are 6 image saliency objects The detection data set (see Table 1), 2 video eye positioning point detection data sets (see Table 2), and the introduction of the 8 data sets are shown in Table 1 and Table 2.
[0079] Table 1
[0080] data set
MSRA
THUS
THURS
DUT-OMRON
DUTS
ECSSD
size
1000
10000
6232
5168
15572
1000
[0081] Table 2
[0082]
[0083]
[0084] Among them, the six image salient object detection data sets of MSRA, THUS,...
Embodiment 3
[0185] Below in conjunction with concrete experimental data, the scheme in embodiment 1 and 2 is carried out feasibility verification, see the following description for details:
[0186] see Figure 7 , i) is the original data frame, (ii) is the model prediction probability map, and (iii) is the visualized heat map.
[0187] Among them, (ii) is the prediction result of the eye positioning point obtained by using the model SGF (E) in the present invention to detect the original data frame in (i), (iii) is the result obtained by the model detection (ii ) is the heatmap obtained after visualization using a color distribution matrix.
PUM
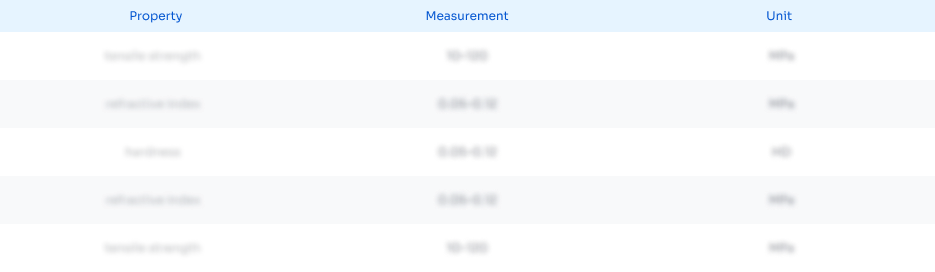
Abstract
Description
Claims
Application Information
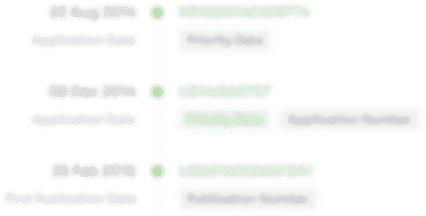
- R&D
- Intellectual Property
- Life Sciences
- Materials
- Tech Scout
- Unparalleled Data Quality
- Higher Quality Content
- 60% Fewer Hallucinations
Browse by: Latest US Patents, China's latest patents, Technical Efficacy Thesaurus, Application Domain, Technology Topic, Popular Technical Reports.
© 2025 PatSnap. All rights reserved.Legal|Privacy policy|Modern Slavery Act Transparency Statement|Sitemap|About US| Contact US: help@patsnap.com