Adaptive stock prediction method of hidden Markov model based on multi-characteristic factor
A prediction method, multi-feature technology, used in data processing applications, character and pattern recognition, instrumentation, etc.
- Summary
- Abstract
- Description
- Claims
- Application Information
AI Technical Summary
Problems solved by technology
Method used
Image
Examples
Embodiment Construction
[0044] The present invention will be further described below in conjunction with the accompanying drawings and specific embodiments.
[0045] The present invention mainly consists of stock data and HMM. The stock data is the trading day data of the Shanghai and Shenzhen Index from 2007.1.4-2017.4.10. The algorithm is an HMM that assumes first-order Markov and the independence of the state and observation values at the current time point.
[0046] S1 data preparation:
[0047] Step 1: Collect data through Caijing.com, Scapy, and indicator calculation formulas, such as Image 6 shown.
[0048] Step 2: Perform preprocessing such as normalization and regularization on the collected data.
[0049] Step 3: Divide the preprocessed collected stock data into a training data set and a testing data set.
[0050] S2 build model parameters:
[0051] Step 1: Use python's hmmlearn.hmm to learn the internal parameters of the hmm algorithm, which is the core algorithm of this model, usi...
PUM
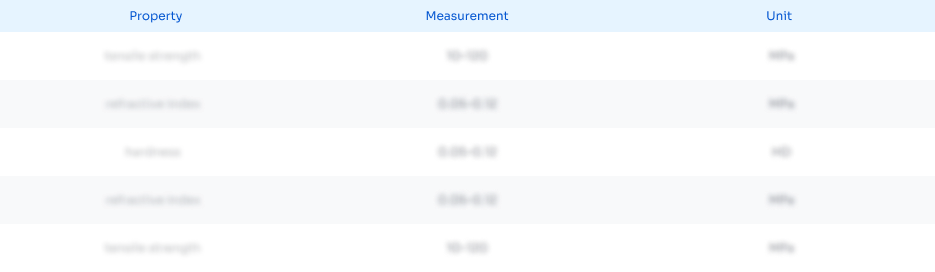
Abstract
Description
Claims
Application Information
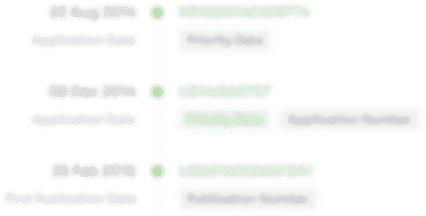
- R&D Engineer
- R&D Manager
- IP Professional
- Industry Leading Data Capabilities
- Powerful AI technology
- Patent DNA Extraction
Browse by: Latest US Patents, China's latest patents, Technical Efficacy Thesaurus, Application Domain, Technology Topic, Popular Technical Reports.
© 2024 PatSnap. All rights reserved.Legal|Privacy policy|Modern Slavery Act Transparency Statement|Sitemap|About US| Contact US: help@patsnap.com