Street front order event video detection method based on deep learning and motion consistency
A technology of deep learning and video detection, applied in computer parts, instruments, character and pattern recognition, etc., can solve the problems that traditional algorithms are difficult to meet the needs, various event forms, event false detection and missed detection, etc.
- Summary
- Abstract
- Description
- Claims
- Application Information
AI Technical Summary
Problems solved by technology
Method used
Image
Examples
Embodiment Construction
[0062] The present invention is based on the video detection method of street order events based on deep learning and motion consistency, and its method steps are:
[0063] 1) Algorithm framework:
[0064] The algorithm framework is set to polling mode, cyclically accessing the front-end video stream, collecting N frames and buffering them in the designated memory, then switching to the next video stream and buffering them in the corresponding memory space. When the internal algorithm function module thread needs to be processed, it is copied from the corresponding memory to the internal cache of the algorithm. After the processing is completed, the running results are uniformly sent to the event judgment thread for final event judgment, and then the memory data where the next video stream is located is copied, using the same way to deal with. This mode can not only ensure that the latest data collected by the current device is processed by the algorithm (the video stream is ...
PUM
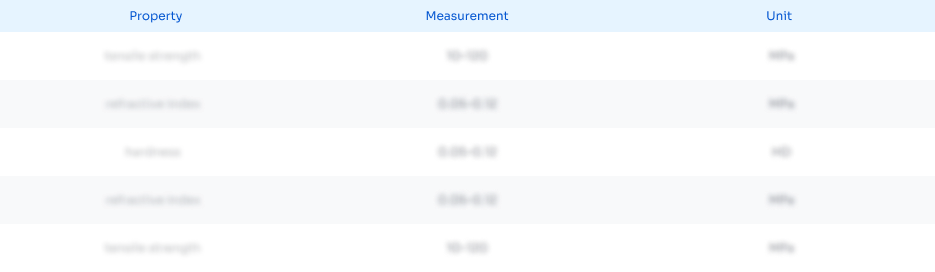
Abstract
Description
Claims
Application Information
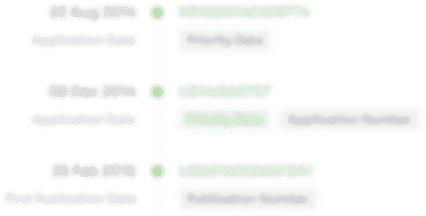
- R&D Engineer
- R&D Manager
- IP Professional
- Industry Leading Data Capabilities
- Powerful AI technology
- Patent DNA Extraction
Browse by: Latest US Patents, China's latest patents, Technical Efficacy Thesaurus, Application Domain, Technology Topic, Popular Technical Reports.
© 2024 PatSnap. All rights reserved.Legal|Privacy policy|Modern Slavery Act Transparency Statement|Sitemap|About US| Contact US: help@patsnap.com