Improved spectral clustering and parallelization method
A spectral clustering and clustering technology, applied in the field of unsupervised learning, which can solve problems such as intractable data
- Summary
- Abstract
- Description
- Claims
- Application Information
AI Technical Summary
Problems solved by technology
Method used
Image
Examples
Embodiment Construction
[0014] Selecting the eigenvectors corresponding to the first 2k largest eigenvalues of the Laplacian matrix as the source data for clustering can not only produce results that are higher than the clustering accuracy of the ordinary NJW algorithm, but also produce results that are lower than the clustering accuracy of the ordinary NJW algorithm. The result of high rate, the volatility of its multiple clustering results is higher than that of ordinary NJW algorithm clustering results. The volatility of multiple clustering accuracy is due to the feature sample space in spectral clustering, and the low-dimensional space samples are more compact. After expanding from k-dimensional to 2k-dimensional, the introduction of a dimension with poor clustering ability increases the The irrelevance between the data makes the data points more dispersed compared with the low-dimensional space; and because the spectral clustering algorithm also uses random initialization center points, it incr...
PUM
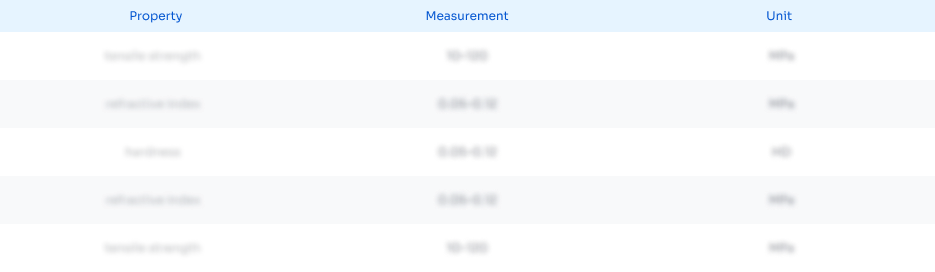
Abstract
Description
Claims
Application Information
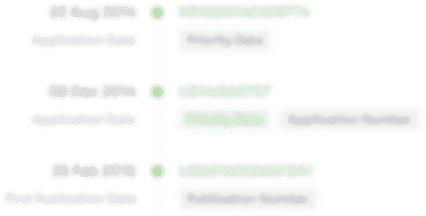
- R&D
- Intellectual Property
- Life Sciences
- Materials
- Tech Scout
- Unparalleled Data Quality
- Higher Quality Content
- 60% Fewer Hallucinations
Browse by: Latest US Patents, China's latest patents, Technical Efficacy Thesaurus, Application Domain, Technology Topic, Popular Technical Reports.
© 2025 PatSnap. All rights reserved.Legal|Privacy policy|Modern Slavery Act Transparency Statement|Sitemap|About US| Contact US: help@patsnap.com