Short-term load prediction method based on variant selection and Gaussian process regression
A Gaussian process regression and short-term load forecasting technology, applied in the field of power system, can solve the problems that the optimization performance is greatly affected by the selection of the initial value, the number of iterations is difficult to determine, and the accuracy of the prediction results is not high, so as to improve the accuracy and generalization ability, The effect of improving model prediction performance
- Summary
- Abstract
- Description
- Claims
- Application Information
AI Technical Summary
Problems solved by technology
Method used
Image
Examples
Embodiment Construction
[0018] Below in conjunction with specific embodiment, further illustrate the present invention, should be understood that these embodiments are only used to illustrate the present invention and are not intended to limit the scope of the present invention, after having read the present invention, those skilled in the art will understand various equivalent forms of the present invention All modifications fall within the scope defined by the appended claims of the present application.
[0019] The idea of the present invention is to use the random forest algorithm in the input variable selection of the short-term load forecasting modeling of the power system, and use the random forest algorithm to give the importance score and sorting of each input variable, thereby combining the Gaussian process regression model and based on the sequence forward The search strategy determines the optimal set of input variables, and the minimum prediction error corresponds to the optimal set of ...
PUM
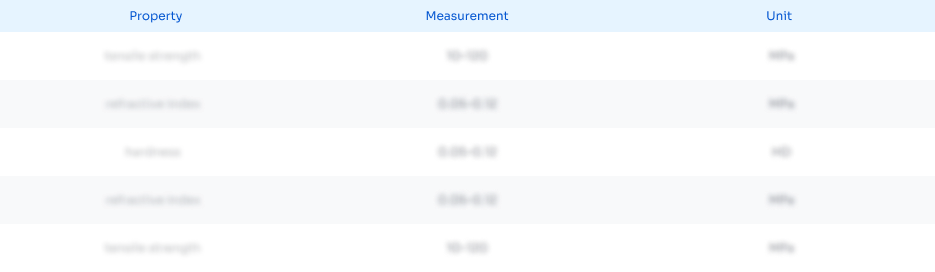
Abstract
Description
Claims
Application Information
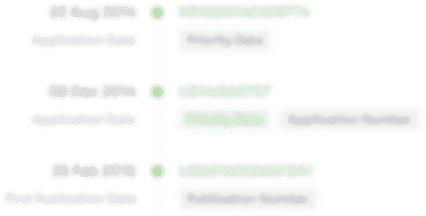
- R&D Engineer
- R&D Manager
- IP Professional
- Industry Leading Data Capabilities
- Powerful AI technology
- Patent DNA Extraction
Browse by: Latest US Patents, China's latest patents, Technical Efficacy Thesaurus, Application Domain, Technology Topic, Popular Technical Reports.
© 2024 PatSnap. All rights reserved.Legal|Privacy policy|Modern Slavery Act Transparency Statement|Sitemap|About US| Contact US: help@patsnap.com