Low-quality face comparison method based on deep convolution neural network
A deep convolution and neural network technology, applied in the field of face comparison, can solve the problems of reducing the flexibility of face comparison technology and limiting the application range of face comparison technology, achieving both accuracy and high efficiency of face comparison Comparison, the effect of less computing resources
- Summary
- Abstract
- Description
- Claims
- Application Information
AI Technical Summary
Problems solved by technology
Method used
Image
Examples
Embodiment 1
[0027] This embodiment proposes a high-efficiency comparison method for low-quality faces based on deep convolutional neural networks, see figure 1 . The specific process is as follows:
[0028] S101: Establish a face training database, establish a low-quality face verification database, and establish a low-quality face test database;
[0029] S102: Normalize each image in the face training database, and input the processed data tensor into the constructed deep convolutional neural network plus fully connected layer to extract features;
[0030] S103: Through the spherical loss function, use the gradient descent method to find the weight value in the fully connected layer and the weight value of each filter in the deep convolutional neural network. During the training process, record the statistics of the low-quality face recognition verification database at the same time. result
[0031] S104: After the training is completed, select the deep convolutional neural network mo...
Embodiment 2
[0076] This embodiment makes some adjustments and extensions to the scheme in embodiment 1, see image 3 :
[0077] Step 201: Using the network structure in Embodiment 1, set the number of filters of the input layer of the convolutional layer of the second stage of the network and the convolutional layer of the residual unit class to 128, and set the input of the convolutional layer of the third stage of the network The number of filters of the convolutional layer of the layer and the residual unit class is 256, and the number of filters of the convolutional layer input layer of the fourth stage of the network and the convolutional layer of the residual unit class is set to 512;
[0078]Step 202: add a convolution adaptation layer after the ResNet network structure, the parameters of the convolution adaptation layer are filter kernel size 3x3, the number of filters is 256, and the convolution step length is 1, abbreviated as k(3,3), 256,s1;
[0079] Step 203: Connect the net...
PUM
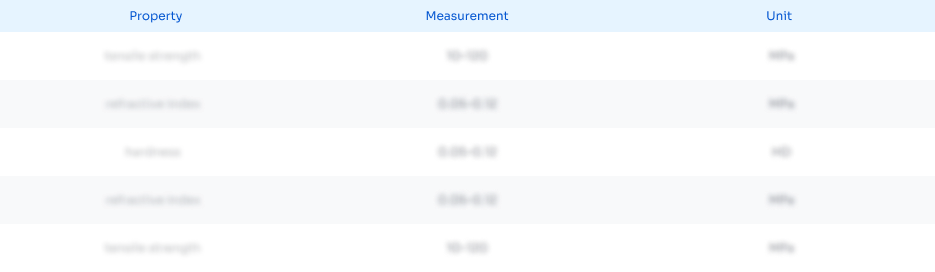
Abstract
Description
Claims
Application Information
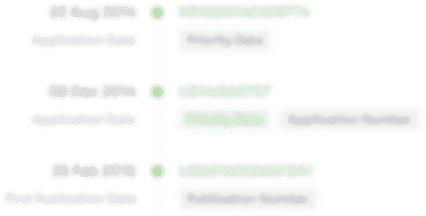
- R&D
- Intellectual Property
- Life Sciences
- Materials
- Tech Scout
- Unparalleled Data Quality
- Higher Quality Content
- 60% Fewer Hallucinations
Browse by: Latest US Patents, China's latest patents, Technical Efficacy Thesaurus, Application Domain, Technology Topic, Popular Technical Reports.
© 2025 PatSnap. All rights reserved.Legal|Privacy policy|Modern Slavery Act Transparency Statement|Sitemap|About US| Contact US: help@patsnap.com