Image quantitative analysis method
An analysis method and image quantification technology, applied in the field of image processing and machine learning applications, can solve problems such as limited resolution and inability to achieve human analysis, and achieve the effect of intuitive abnormal areas, intuitive discrimination, and enhancement of abnormal areas
- Summary
- Abstract
- Description
- Claims
- Application Information
AI Technical Summary
Problems solved by technology
Method used
Image
Examples
Embodiment 1
[0022] Embodiment 1: as figure 1 Shown, a kind of image quantitative analysis method comprises step 01)-step 02):
[0023] Step 01) Acquire raw image samples. In this step 01), the original image samples include original training samples and original test samples. To meet the needs of the following steps, in this embodiment, the original training samples are divided into training set samples and verification set samples. For example, the original image samples used in this embodiment are MRI images of T1 and PD modalities, the image resolution is 0.3mm×0.3mm×1mm, and the image size is 480mm×480mm×24mm. The data precision of the original training sample and the original test sample is adjusted to single and stored in .hdf5 format. The original training samples can be MRI images of T1 and PD modalities of healthy people. Characteristic regions of the cartilage-bone joint of the knee in this MRI image.
[0024]Step 02) Smooth blurring. Lesion area smooth blur is used to blu...
Embodiment 2
[0029] Embodiment 2. The difference between this embodiment and Embodiment 1 is that the smoothing and blurring processing steps adopted in this embodiment are different from those in Embodiment 1. Specifically, this embodiment adopts step 202 to describe this in detail:
[0030] Step 022): In the smoothing and blurring processing step, normalization processing is included: the mat2gray function in MATLAB is used to carry out normalization processing to the original image sample, so that the output image data interval is set to [0,1]; in this original The center of the image sample acquires a preset size area, and the pixel average value of the preset size area is used as the pixel value of the original image sample.
[0031] Based on the training samples processed in step 202 above, the subsequent steps are also different. For example, in step 03), the super-resolution reconstruction network structure can be shared, but the respective network parameters need to be trained sep...
PUM
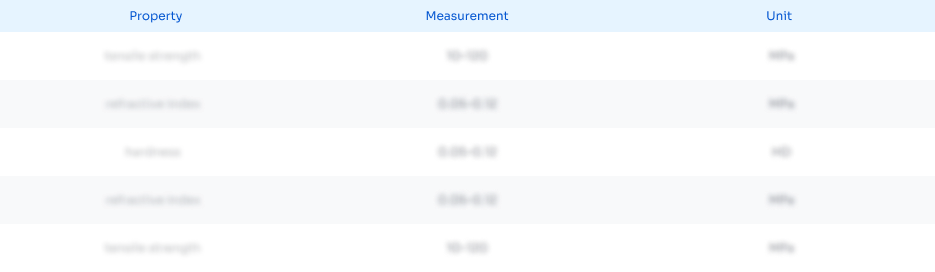
Abstract
Description
Claims
Application Information
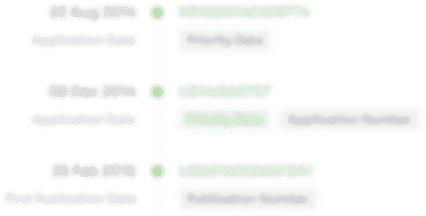
- R&D Engineer
- R&D Manager
- IP Professional
- Industry Leading Data Capabilities
- Powerful AI technology
- Patent DNA Extraction
Browse by: Latest US Patents, China's latest patents, Technical Efficacy Thesaurus, Application Domain, Technology Topic, Popular Technical Reports.
© 2024 PatSnap. All rights reserved.Legal|Privacy policy|Modern Slavery Act Transparency Statement|Sitemap|About US| Contact US: help@patsnap.com